using PharmaDatasets
using DataFramesMeta
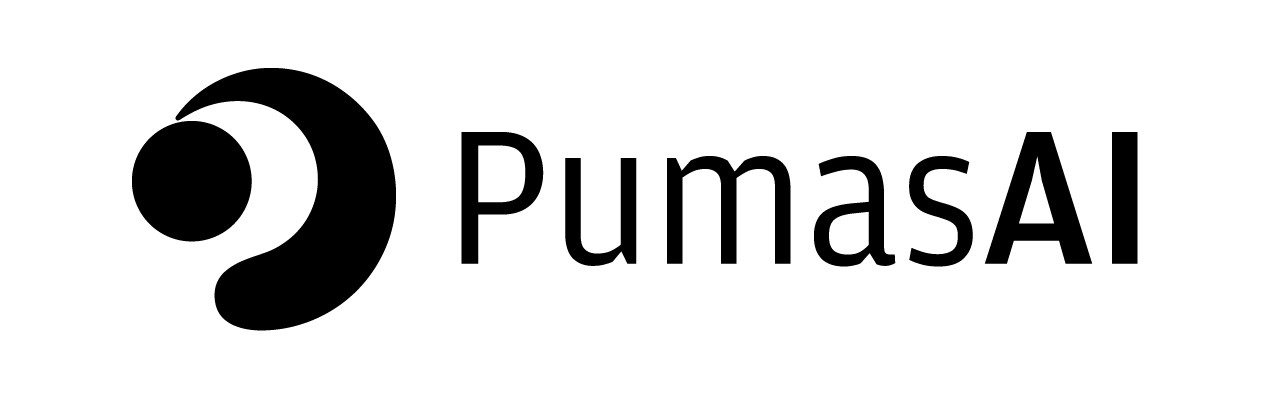
Tutorial 7 - Reshaping DataFrame
s
Data comes in many forms, but some forms are more amenable to work with than others. This tutorial provides an introduction to some common patterns for reshaping data tables to make them easier to work with.
1 ↔︎️ Wide vs ↕️ Long form
One of the more common distinctions in tabular data, is between “wide” form, in which each row represents all related observations for a single specimen, site, or measurement period, or “long” form, where each row represents a single observation, and only enough columns as necessary to uniquely identify the observation type and the thing it’s tied to.
For example, imagine that you are collecting blood pressure measurements from a group of subjects over time. Your data table in wide form might look like:
Subject | date | systolic | diastolic | heart rate |
---|---|---|---|---|
1 | 2022-01-03 | 120 | 80 | 65 |
1 | 2022-01-05 | 135 | 90 | 68 |
2 | 2022-01-10 | 115 | 84 | 72 |
whereas the same data in long form would look like:
Subject | date | variable | value |
---|---|---|---|
1 | 2022-01-03 | systolic | 120 |
1 | 2022-01-03 | diastolic | 80 |
1 | 2022-01-03 | heart rate | 65 |
1 | 2022-01-05 | systolic | 135 |
1 | 2022-01-05 | diastolic | 90 |
1 | 2022-01-05 | heart rate | 68 |
2 | 2022-01-10 | systolic | 115 |
2 | 2022-01-10 | diastolic | 84 |
2 | 2022-01-10 | heart rate | 72 |
If you collect additional types of data, in the wide table, you’d add additional columns, while in the long table you’d add additional rows.
Each form has its uses, and happily, you don’t have to pick just one - it’s easy to switch between them depending on your needs. Let’s load some actual data to see how this works.
= dataset("pumas_tutorials/wide_data") wide
Row | IDS | 0.0 | 30 min | 1 hrs | 2 hrs | 4 hrs |
---|---|---|---|---|---|---|
String15 | Float64 | Float64 | Float64 | Float64 | Float64 | |
1 | ID001_S001 | 17.9 | 16.5 | 15.5 | 10.2 | 17.4 |
2 | ID001_S002 | 11.9 | 13.7 | 11.7 | 16.0 | 16.9 |
3 | ID001_S003 | 14.5 | 11.0 | 17.4 | 10.4 | 14.1 |
4 | ID002_S001 | 18.9 | 18.3 | 13.6 | 16.8 | 18.2 |
5 | ID002_S002 | 12.1 | 11.8 | 11.5 | 12.6 | 17.8 |
6 | ID002_S003 | 10.5 | 17.4 | 18.3 | 19.7 | 18.3 |
7 | ID003_S001 | 18.4 | 18.4 | 14.9 | 13.4 | 18.0 |
8 | ID003_S002 | 13.1 | 17.2 | 10.6 | 10.2 | 17.5 |
9 | ID003_S003 | 18.5 | 10.1 | 19.1 | 10.1 | 15.2 |
10 | ID004_S001 | 15.3 | 18.9 | 18.3 | 15.5 | 17.2 |
11 | ID004_S002 | 10.5 | 18.9 | 10.3 | 15.6 | 15.9 |
12 | ID004_S003 | 16.4 | 19.2 | 15.5 | 19.1 | 17.6 |
13 | ID005_S001 | 11.1 | 16.1 | 18.0 | 19.2 | 17.7 |
14 | ID005_S002 | 12.4 | 16.7 | 11.9 | 13.0 | 14.4 |
15 | ID005_S003 | 10.2 | 11.4 | 15.3 | 19.9 | 13.8 |
And we’ll also split the “IDS” column into its component parts.
@rtransform! wide @astable begin
= split(:IDS, '_')
(id, s) :id = id
:sid = s
end
first(wide, 5)
Row | IDS | 0.0 | 30 min | 1 hrs | 2 hrs | 4 hrs | id | sid |
---|---|---|---|---|---|---|---|---|
String15 | Float64 | Float64 | Float64 | Float64 | Float64 | SubStrin… | SubStrin… | |
1 | ID001_S001 | 17.9 | 16.5 | 15.5 | 10.2 | 17.4 | ID001 | S001 |
2 | ID001_S002 | 11.9 | 13.7 | 11.7 | 16.0 | 16.9 | ID001 | S002 |
3 | ID001_S003 | 14.5 | 11.0 | 17.4 | 10.4 | 14.1 | ID001 | S003 |
4 | ID002_S001 | 18.9 | 18.3 | 13.6 | 16.8 | 18.2 | ID002 | S001 |
5 | ID002_S002 | 12.1 | 11.8 | 11.5 | 12.6 | 17.8 | ID002 | S002 |
# reorder them...
select!(wide, :id, :sid, Not(:IDS))
Row | id | sid | 0.0 | 30 min | 1 hrs | 2 hrs | 4 hrs |
---|---|---|---|---|---|---|---|
SubStrin… | SubStrin… | Float64 | Float64 | Float64 | Float64 | Float64 | |
1 | ID001 | S001 | 17.9 | 16.5 | 15.5 | 10.2 | 17.4 |
2 | ID001 | S002 | 11.9 | 13.7 | 11.7 | 16.0 | 16.9 |
3 | ID001 | S003 | 14.5 | 11.0 | 17.4 | 10.4 | 14.1 |
4 | ID002 | S001 | 18.9 | 18.3 | 13.6 | 16.8 | 18.2 |
5 | ID002 | S002 | 12.1 | 11.8 | 11.5 | 12.6 | 17.8 |
6 | ID002 | S003 | 10.5 | 17.4 | 18.3 | 19.7 | 18.3 |
7 | ID003 | S001 | 18.4 | 18.4 | 14.9 | 13.4 | 18.0 |
8 | ID003 | S002 | 13.1 | 17.2 | 10.6 | 10.2 | 17.5 |
9 | ID003 | S003 | 18.5 | 10.1 | 19.1 | 10.1 | 15.2 |
10 | ID004 | S001 | 15.3 | 18.9 | 18.3 | 15.5 | 17.2 |
11 | ID004 | S002 | 10.5 | 18.9 | 10.3 | 15.6 | 15.9 |
12 | ID004 | S003 | 16.4 | 19.2 | 15.5 | 19.1 | 17.6 |
13 | ID005 | S001 | 11.1 | 16.1 | 18.0 | 19.2 | 17.7 |
14 | ID005 | S002 | 12.4 | 16.7 | 11.9 | 13.0 | 14.4 |
15 | ID005 | S003 | 10.2 | 11.4 | 15.3 | 19.9 | 13.8 |
2 🥞 Stack
We use the stack()
function to go from wide-form to long-form. The columns that represent particular observations are called “measurement variables” and those that uniquely identify each observation are the “ID variables.”
If we only pass the DataFrame
, by default, stack()
will treat any floating point column as a measurement var, and all other columns as id vars. In this case, that’s actually what we want:
= stack(wide) long
Row | id | sid | variable | value |
---|---|---|---|---|
SubStrin… | SubStrin… | String | Float64 | |
1 | ID001 | S001 | 0.0 | 17.9 |
2 | ID001 | S002 | 0.0 | 11.9 |
3 | ID001 | S003 | 0.0 | 14.5 |
4 | ID002 | S001 | 0.0 | 18.9 |
5 | ID002 | S002 | 0.0 | 12.1 |
6 | ID002 | S003 | 0.0 | 10.5 |
7 | ID003 | S001 | 0.0 | 18.4 |
8 | ID003 | S002 | 0.0 | 13.1 |
9 | ID003 | S003 | 0.0 | 18.5 |
10 | ID004 | S001 | 0.0 | 15.3 |
11 | ID004 | S002 | 0.0 | 10.5 |
12 | ID004 | S003 | 0.0 | 16.4 |
13 | ID005 | S001 | 0.0 | 11.1 |
⋮ | ⋮ | ⋮ | ⋮ | ⋮ |
64 | ID002 | S001 | 4 hrs | 18.2 |
65 | ID002 | S002 | 4 hrs | 17.8 |
66 | ID002 | S003 | 4 hrs | 18.3 |
67 | ID003 | S001 | 4 hrs | 18.0 |
68 | ID003 | S002 | 4 hrs | 17.5 |
69 | ID003 | S003 | 4 hrs | 15.2 |
70 | ID004 | S001 | 4 hrs | 17.2 |
71 | ID004 | S002 | 4 hrs | 15.9 |
72 | ID004 | S003 | 4 hrs | 17.6 |
73 | ID005 | S001 | 4 hrs | 17.7 |
74 | ID005 | S002 | 4 hrs | 14.4 |
75 | ID005 | S003 | 4 hrs | 13.8 |
Alternatively, we can explicitly pass the measurement vars and the id vars as the second and third positional arguments respectively.
stack(wide, [Symbol("0.0"), Symbol("30 min")], [:id, :sid])
Row | id | sid | variable | value |
---|---|---|---|---|
SubStrin… | SubStrin… | String | Float64 | |
1 | ID001 | S001 | 0.0 | 17.9 |
2 | ID001 | S002 | 0.0 | 11.9 |
3 | ID001 | S003 | 0.0 | 14.5 |
4 | ID002 | S001 | 0.0 | 18.9 |
5 | ID002 | S002 | 0.0 | 12.1 |
6 | ID002 | S003 | 0.0 | 10.5 |
7 | ID003 | S001 | 0.0 | 18.4 |
8 | ID003 | S002 | 0.0 | 13.1 |
9 | ID003 | S003 | 0.0 | 18.5 |
10 | ID004 | S001 | 0.0 | 15.3 |
11 | ID004 | S002 | 0.0 | 10.5 |
12 | ID004 | S003 | 0.0 | 16.4 |
13 | ID005 | S001 | 0.0 | 11.1 |
⋮ | ⋮ | ⋮ | ⋮ | ⋮ |
19 | ID002 | S001 | 30 min | 18.3 |
20 | ID002 | S002 | 30 min | 11.8 |
21 | ID002 | S003 | 30 min | 17.4 |
22 | ID003 | S001 | 30 min | 18.4 |
23 | ID003 | S002 | 30 min | 17.2 |
24 | ID003 | S003 | 30 min | 10.1 |
25 | ID004 | S001 | 30 min | 18.9 |
26 | ID004 | S002 | 30 min | 18.9 |
27 | ID004 | S003 | 30 min | 19.2 |
28 | ID005 | S001 | 30 min | 16.1 |
29 | ID005 | S002 | 30 min | 16.7 |
30 | ID005 | S003 | 30 min | 11.4 |
As you can see, stack()
gives the new observation columns the names :variable
and :value
, but we can change this with the variable_name
and value_name
keyword arguments:
= stack(wide; variable_name = :original, value_name = :observed) long_rename
Row | id | sid | original | observed |
---|---|---|---|---|
SubStrin… | SubStrin… | String | Float64 | |
1 | ID001 | S001 | 0.0 | 17.9 |
2 | ID001 | S002 | 0.0 | 11.9 |
3 | ID001 | S003 | 0.0 | 14.5 |
4 | ID002 | S001 | 0.0 | 18.9 |
5 | ID002 | S002 | 0.0 | 12.1 |
6 | ID002 | S003 | 0.0 | 10.5 |
7 | ID003 | S001 | 0.0 | 18.4 |
8 | ID003 | S002 | 0.0 | 13.1 |
9 | ID003 | S003 | 0.0 | 18.5 |
10 | ID004 | S001 | 0.0 | 15.3 |
11 | ID004 | S002 | 0.0 | 10.5 |
12 | ID004 | S003 | 0.0 | 16.4 |
13 | ID005 | S001 | 0.0 | 11.1 |
⋮ | ⋮ | ⋮ | ⋮ | ⋮ |
64 | ID002 | S001 | 4 hrs | 18.2 |
65 | ID002 | S002 | 4 hrs | 17.8 |
66 | ID002 | S003 | 4 hrs | 18.3 |
67 | ID003 | S001 | 4 hrs | 18.0 |
68 | ID003 | S002 | 4 hrs | 17.5 |
69 | ID003 | S003 | 4 hrs | 15.2 |
70 | ID004 | S001 | 4 hrs | 17.2 |
71 | ID004 | S002 | 4 hrs | 15.9 |
72 | ID004 | S003 | 4 hrs | 17.6 |
73 | ID005 | S001 | 4 hrs | 17.7 |
74 | ID005 | S002 | 4 hrs | 14.4 |
75 | ID005 | S003 | 4 hrs | 13.8 |
3 🏠️ 🃏 Unstack
Of course, sometimes we may want to go the other way. Given a long-form DataFrame
, the unstack()
function can be used to convert to wide form.
unstack(long)
Row | id | sid | 0.0 | 30 min | 1 hrs | 2 hrs | 4 hrs |
---|---|---|---|---|---|---|---|
SubStrin… | SubStrin… | Float64? | Float64? | Float64? | Float64? | Float64? | |
1 | ID001 | S001 | 17.9 | 16.5 | 15.5 | 10.2 | 17.4 |
2 | ID001 | S002 | 11.9 | 13.7 | 11.7 | 16.0 | 16.9 |
3 | ID001 | S003 | 14.5 | 11.0 | 17.4 | 10.4 | 14.1 |
4 | ID002 | S001 | 18.9 | 18.3 | 13.6 | 16.8 | 18.2 |
5 | ID002 | S002 | 12.1 | 11.8 | 11.5 | 12.6 | 17.8 |
6 | ID002 | S003 | 10.5 | 17.4 | 18.3 | 19.7 | 18.3 |
7 | ID003 | S001 | 18.4 | 18.4 | 14.9 | 13.4 | 18.0 |
8 | ID003 | S002 | 13.1 | 17.2 | 10.6 | 10.2 | 17.5 |
9 | ID003 | S003 | 18.5 | 10.1 | 19.1 | 10.1 | 15.2 |
10 | ID004 | S001 | 15.3 | 18.9 | 18.3 | 15.5 | 17.2 |
11 | ID004 | S002 | 10.5 | 18.9 | 10.3 | 15.6 | 15.9 |
12 | ID004 | S003 | 16.4 | 19.2 | 15.5 | 19.1 | 17.6 |
13 | ID005 | S001 | 11.1 | 16.1 | 18.0 | 19.2 | 17.7 |
14 | ID005 | S002 | 12.4 | 16.7 | 11.9 | 13.0 | 14.4 |
15 | ID005 | S003 | 10.2 | 11.4 | 15.3 | 19.9 | 13.8 |
If your observation columns are named something other than :variable
and :value
, you’ll need to tell unstack()
which columns to use.
unstack(long_rename, :original, :observed)
Row | id | sid | 0.0 | 30 min | 1 hrs | 2 hrs | 4 hrs |
---|---|---|---|---|---|---|---|
SubStrin… | SubStrin… | Float64? | Float64? | Float64? | Float64? | Float64? | |
1 | ID001 | S001 | 17.9 | 16.5 | 15.5 | 10.2 | 17.4 |
2 | ID001 | S002 | 11.9 | 13.7 | 11.7 | 16.0 | 16.9 |
3 | ID001 | S003 | 14.5 | 11.0 | 17.4 | 10.4 | 14.1 |
4 | ID002 | S001 | 18.9 | 18.3 | 13.6 | 16.8 | 18.2 |
5 | ID002 | S002 | 12.1 | 11.8 | 11.5 | 12.6 | 17.8 |
6 | ID002 | S003 | 10.5 | 17.4 | 18.3 | 19.7 | 18.3 |
7 | ID003 | S001 | 18.4 | 18.4 | 14.9 | 13.4 | 18.0 |
8 | ID003 | S002 | 13.1 | 17.2 | 10.6 | 10.2 | 17.5 |
9 | ID003 | S003 | 18.5 | 10.1 | 19.1 | 10.1 | 15.2 |
10 | ID004 | S001 | 15.3 | 18.9 | 18.3 | 15.5 | 17.2 |
11 | ID004 | S002 | 10.5 | 18.9 | 10.3 | 15.6 | 15.9 |
12 | ID004 | S003 | 16.4 | 19.2 | 15.5 | 19.1 | 17.6 |
13 | ID005 | S001 | 11.1 | 16.1 | 18.0 | 19.2 | 17.7 |
14 | ID005 | S002 | 12.4 | 16.7 | 11.9 | 13.0 | 14.4 |
15 | ID005 | S003 | 10.2 | 11.4 | 15.3 | 19.9 | 13.8 |
Any column in the long-form table that is not the variable
or value
column will be assumed to be one of the unique identifiers. If this is not the case, you may provide the unique id column(s) as an additional argument. But, if the column(s) supplied are not actually unique, unstack()
will throw an error.
unstack(long_rename, :id, :original, :observed)
ArgumentError: Duplicate entries in unstack at row 2 for key ("ID001",) and variable 0.0. Pass `combine` keyword argument to specify how they should be handled. Stacktrace: [1] _unstack(df::DataFrame, rowkeys::Vector{Int64}, colkey::Int64, g_colkey::GroupedDataFrame{DataFrame}, valuecol::Vector{Float64}, g_rowkey::GroupedDataFrame{DataFrame}, renamecols::typeof(identity), allowmissing::Bool, noduplicates::Bool, fill::Missing) @ DataFrames ~/run/_work/PumasTutorials.jl/PumasTutorials.jl/custom_julia_depot/packages/DataFrames/kcA9R/src/abstractdataframe/reshape.jl:569 [2] unstack(df::DataFrame, rowkeys::Symbol, colkey::Symbol, values::Symbol; renamecols::Function, allowmissing::Bool, allowduplicates::Bool, combine::Function, fill::Missing, threads::Bool) @ DataFrames ~/run/_work/PumasTutorials.jl/PumasTutorials.jl/custom_julia_depot/packages/DataFrames/kcA9R/src/abstractdataframe/reshape.jl:478 [3] unstack(df::DataFrame, rowkeys::Symbol, colkey::Symbol, values::Symbol) @ DataFrames ~/run/_work/PumasTutorials.jl/PumasTutorials.jl/custom_julia_depot/packages/DataFrames/kcA9R/src/abstractdataframe/reshape.jl:420 [4] top-level scope @ ~/run/_work/PumasTutorials.jl/PumasTutorials.jl/tutorials/DataWranglingInJulia/07-pivoting.qmd:207
If the provided column(s) are not unique identifiers, you can specify with the combine
keyword argument which of the duplicate rows you want to keep. Its value must be a function that is for each combination of identifiers called on a vector view of all corresponding rows. By setting it to last
, only the last values encountered will be retained.
unstack(long_rename, :id, :original, :observed; combine = last)
Row | id | 0.0 | 30 min | 1 hrs | 2 hrs | 4 hrs |
---|---|---|---|---|---|---|
SubStrin… | Float64? | Float64? | Float64? | Float64? | Float64? | |
1 | ID001 | 14.5 | 11.0 | 17.4 | 10.4 | 14.1 |
2 | ID002 | 10.5 | 17.4 | 18.3 | 19.7 | 18.3 |
3 | ID003 | 18.5 | 10.1 | 19.1 | 10.1 | 15.2 |
4 | ID004 | 16.4 | 19.2 | 15.5 | 19.1 | 17.6 |
5 | ID005 | 10.2 | 11.4 | 15.3 | 19.9 | 13.8 |
Similarly, by setting it to first
, only the first values encountered will be retained.
unstack(long_rename, :id, :original, :observed; combine = first)
Row | id | 0.0 | 30 min | 1 hrs | 2 hrs | 4 hrs |
---|---|---|---|---|---|---|
SubStrin… | Float64? | Float64? | Float64? | Float64? | Float64? | |
1 | ID001 | 17.9 | 16.5 | 15.5 | 10.2 | 17.4 |
2 | ID002 | 18.9 | 18.3 | 13.6 | 16.8 | 18.2 |
3 | ID003 | 18.4 | 18.4 | 14.9 | 13.4 | 18.0 |
4 | ID004 | 15.3 | 18.9 | 18.3 | 15.5 | 17.2 |
5 | ID005 | 11.1 | 16.1 | 18.0 | 19.2 | 17.7 |
4 🥠 Split, Apply, Combine
A common pattern in data analysis is to split a dataset into groups, perform some calculations within those groups, and then combine the results into a new table. Combining reshaping and the transformations we learned earlier makes this trivial.
For example:
using Statistics
stack(wide, [:id, :sid])
Row | 0.0 | 30 min | 1 hrs | 2 hrs | 4 hrs | variable | value |
---|---|---|---|---|---|---|---|
Float64 | Float64 | Float64 | Float64 | Float64 | String | SubStrin… | |
1 | 17.9 | 16.5 | 15.5 | 10.2 | 17.4 | id | ID001 |
2 | 11.9 | 13.7 | 11.7 | 16.0 | 16.9 | id | ID001 |
3 | 14.5 | 11.0 | 17.4 | 10.4 | 14.1 | id | ID001 |
4 | 18.9 | 18.3 | 13.6 | 16.8 | 18.2 | id | ID002 |
5 | 12.1 | 11.8 | 11.5 | 12.6 | 17.8 | id | ID002 |
6 | 10.5 | 17.4 | 18.3 | 19.7 | 18.3 | id | ID002 |
7 | 18.4 | 18.4 | 14.9 | 13.4 | 18.0 | id | ID003 |
8 | 13.1 | 17.2 | 10.6 | 10.2 | 17.5 | id | ID003 |
9 | 18.5 | 10.1 | 19.1 | 10.1 | 15.2 | id | ID003 |
10 | 15.3 | 18.9 | 18.3 | 15.5 | 17.2 | id | ID004 |
11 | 10.5 | 18.9 | 10.3 | 15.6 | 15.9 | id | ID004 |
12 | 16.4 | 19.2 | 15.5 | 19.1 | 17.6 | id | ID004 |
13 | 11.1 | 16.1 | 18.0 | 19.2 | 17.7 | id | ID005 |
⋮ | ⋮ | ⋮ | ⋮ | ⋮ | ⋮ | ⋮ | ⋮ |
19 | 18.9 | 18.3 | 13.6 | 16.8 | 18.2 | sid | S001 |
20 | 12.1 | 11.8 | 11.5 | 12.6 | 17.8 | sid | S002 |
21 | 10.5 | 17.4 | 18.3 | 19.7 | 18.3 | sid | S003 |
22 | 18.4 | 18.4 | 14.9 | 13.4 | 18.0 | sid | S001 |
23 | 13.1 | 17.2 | 10.6 | 10.2 | 17.5 | sid | S002 |
24 | 18.5 | 10.1 | 19.1 | 10.1 | 15.2 | sid | S003 |
25 | 15.3 | 18.9 | 18.3 | 15.5 | 17.2 | sid | S001 |
26 | 10.5 | 18.9 | 10.3 | 15.6 | 15.9 | sid | S002 |
27 | 16.4 | 19.2 | 15.5 | 19.1 | 17.6 | sid | S003 |
28 | 11.1 | 16.1 | 18.0 | 19.2 | 17.7 | sid | S001 |
29 | 12.4 | 16.7 | 11.9 | 13.0 | 14.4 | sid | S002 |
30 | 10.2 | 11.4 | 15.3 | 19.9 | 13.8 | sid | S003 |
@chain wide begin
stackgroupby(:variable)
@combine(:μ = mean(:value), :σ = std(:value))
end
Row | variable | μ | σ |
---|---|---|---|
String | Float64 | Float64 | |
1 | 0.0 | 14.1133 | 3.21911 |
2 | 30 min | 15.7067 | 3.2168 |
3 | 1 hrs | 14.7933 | 3.04102 |
4 | 2 hrs | 14.78 | 3.66688 |
5 | 4 hrs | 16.6667 | 1.56464 |