using Pumas
using PumasUtilities
using Random
using CairoMakie
using AlgebraOfGraphics
using CSV
using DataFramesMeta
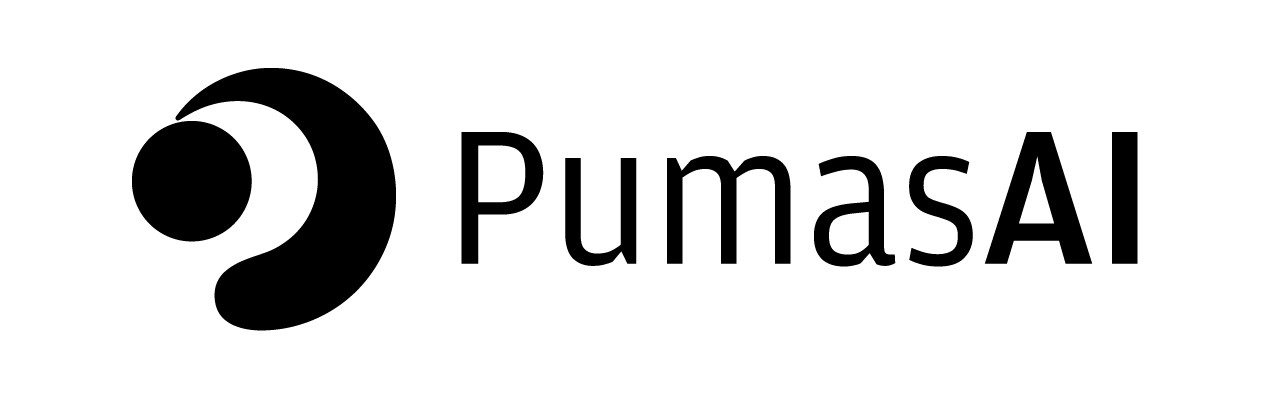
PK43 - Multiple Absorption Routes
1 Learning Outcome
In this exercise, we will be looking at multiple absorption routes and how a model helps in understanding the absorption of sublingually administered doses, partly from the buccal cavity and partly from the gastrointestinal (GI) tract.
2 Objectives
In this model, you will learn how to write a differential equation model for a drug that is partly absorbed from the buccal and partly from the GI and simulate for a single subject.
3 Background
Before constructing a model, it is important to establish the process the model will follow and a scenario for the simulation.
Below is the scenario for this tutorial:
- Structural model - 1 compartment linear elimination with first order absorption
- Route of administration - Sublingual
- Dosage Regimen - 2 mg Sublingual dose
- Number of Subjects - 1
This diagram describes how such an administered dose will be handled, which facilitates building the model.
4 Libraries
Call the required libraries to get started.
5 Model
In this one compartment model, we administer the dose sublingually at 2 sites. Both sites are accounted for in the @dosecontrol
block, including the lag time from the absorption process occurring physiologically in the GI tract.
= @model begin
pk_43 @metadata begin
= "Multiple Absorption Model"
desc = u"hr"
timeu end
@param begin
"""
Absorption rate constant(rapid from buccal) (hr⁻¹)
"""
∈ RealDomain(lower = 0)
tvkar """
Absorption rate constant(delayed from GI) (hr⁻¹)
"""
∈ RealDomain(lower = 0)
tvkad """
Volume of Central Compartment (L)
"""
∈ RealDomain(lower = 0)
tvv """
Lagtime (hr)
"""
∈ RealDomain(lower = 0)
tvlag """
Fraction of drug absorbed
"""
∈ RealDomain(lower = 0)
tvfa """
Elimination rate constant (hr⁻¹)
"""
∈ RealDomain(lower = 0)
tvk ∈ PDiagDomain(4)
Ω """
Additive RUV
"""
∈ RealDomain(lower = 0)
σ_add end
@random begin
~ MvNormal(Ω)
η end
@pre begin
= tvkar * exp(η[1])
KaR = tvkad * exp(η[2])
KaD = tvv * exp(η[3])
V = tvk * exp(η[4])
K end
@dosecontrol begin
= (Buccal = tvfa, Gi = 1 - tvfa)
bioav = (Gi = tvlag,)
lags end
@dynamics begin
' = -KaR * Buccal
Buccal' = -KaD * Gi
Gi' = KaR * Buccal + KaD * Gi - K * Central
Centralend
@derived begin
= @. Central / V
cp """
Observed Concentration (mcg/L)
"""
~ @. Normal(cp, σ_add)
dv end
end
PumasModel
Parameters: tvkar, tvkad, tvv, tvlag, tvfa, tvk, Ω, σ_add
Random effects: η
Covariates:
Dynamical system variables: Buccal, Gi, Central
Dynamical system type: Matrix exponential
Derived: cp, dv
Observed: cp, dv
6 Parameters
Parameters provided for simulation.
Vc
- Volume of Central Compartment (L)K
- Elimination rate constant (hr⁻¹)Kar
- Absorption rate constant(rapid from buccal) (hr⁻¹)Kad
- Absorption rate constant(delayed from GI) (hr⁻¹)Fa
- Fraction of drug absorbedlags
- Lagtime (hr)Ω
- Between Subject Variabilityσ
- Residual error
These are the initial estimates we will be using in this model exercise. Note that tv
represents the typical value for parameters.
= (;
param = 7.62369,
tvkar = 1.0751,
tvkad = 20.6274,
tvv = 0.0886931,
tvk = 0.515023,
tvfa = 2.29614,
tvlag = Diagonal([0.01, 0.01, 0.01, 0.01]),
Ω = 0.86145,
σ_add )
7 Dosage Regimen
To start the simulation process, the dosing regimen specified in the background section must be developed first prior to running a simulation.
The dosage regimen is specified as:
A Single subject receiving a 2 mg dose given orally (absorbed - Sublingually and remaining from the Gut).
This is how to establish the dosing regimen:
= DosageRegimen(2000; time = 0, cmt = [1, 2]) ev1
Row | time | cmt | amt | evid | ii | addl | rate | duration | ss | route |
---|---|---|---|---|---|---|---|---|---|---|
Float64 | Int64 | Float64 | Int8 | Float64 | Int64 | Float64 | Float64 | Int8 | NCA.Route | |
1 | 0.0 | 1 | 2000.0 | 1 | 0.0 | 0 | 0.0 | 0.0 | 0 | NullRoute |
2 | 0.0 | 2 | 2000.0 | 1 | 0.0 | 0 | 0.0 | 0.0 | 0 | NullRoute |
This is how to create the single subject undergoing the dosing regimen above.
= Subject(; id = 1, events = ev1) sub1
Subject
ID: 1
Events: 2
8 Simulation
Let’s simulate plasma concentration with specific observation times after a sublingual dose, considering multiple absorption routes.
Random.seed!()
The Random.seed!
function is included here for purposes of reproducibility of the simulation in this tutorial. Specification of a seed value would not be required in a Pumas workflow that is estimating model parameters.
Random.seed!(123)
= simobs(pk_43, sub1, param, obstimes = 0.00:0.01:24) sim_sub1
SimulatedObservations
Simulated variables: cp, dv
Time: 0.0:0.01:24.0
9 Visualization
From the plot below, the concentration can be observed having a varying absorption profile.
@chain DataFrame(sim_sub1) begin
dropmissing(:cp)
data(_) *
mapping(:time => "Time (minutes)", :cp => "Concentration (μg/L)";) *
visual(Lines; linewidth = 4)
draw(; figure = (; fontsize = 22), axis = (; xticks = 0:5:25))
end
10 Population Simulation
This block updates the parameters of the model to increase intersubject variability in parameters and defines timepoints for prediction of concentrations. The results are written to a CSV file.
= (
par = 7.62369,
tvkar = 1.0751,
tvkad = 20.6274,
tvv = 0.0886931,
tvk = 0.515023,
tvfa = 2.29614,
tvlag = Diagonal([0.0635, 0.0125, 0.03651, 0.0198]),
Ω = 1.86145,
σ_add
)
= DosageRegimen(2000; time = 0, cmt = [1, 2])
ev1 = map(i -> Subject(id = i, events = ev1), 1:68)
pop
Random.seed!(1234)
= simobs(
sim_pop
pk_43,
pop,
par,= [0.1, 0.15, 0.25, 0.5, 0.75, 0.8, 1, 1.25, 1.5, 2, 3, 4, 5, 6, 8, 12, 24],
obstimes
)
= DataFrame(sim_pop)
df_sim #CSV.write("pk_43.csv", df_sim)
With the CSV.write
function, you can input the name of the DataFrame
(df_sim
) and the file name of your choice (pk_43.csv
) to save the file to your local directory or repository.
11 Conclusion
Constructing a model with multiple absorption routes involves:
- understanding the process of how the drug is absorbed and passed through the system,
- translating the absorption and subsequent processes into ODEs in Pumas, and
- simulating the model in a single patient for evaluation.