using Pumas
using PharmaDatasets
using DataFramesMeta
using PumasUtilities
using CSV
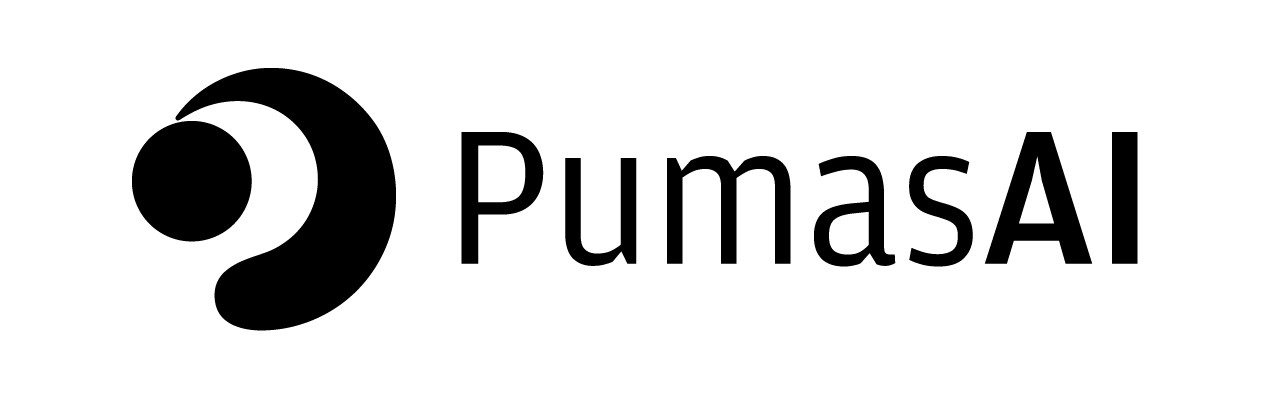
Case Study III: Development of a population PKPD model
1 Introduction
This is the third tutorial in a series of case studies based on the tutorials found here . The third case study is about building a sequential PKPD model. It has IV infusion dosing, PK is governed by a simple one compartment model, and PD is an indirect response model (IDR) of histamine concentrations.
2 Data
The datasets are available from the PharmaDatasets
package. One for the PK model (CS3_IVINFEST
) and another for the PD model (CS3_IVINFPDEST
). First, we read the data for the PK model. We define an :evid
and a :cmt
column and set all event row values of :CONC
to missing
.
= CSV.read(dataset("pumas/event_data/CS3_IVINFEST", String), DataFrame; header = 4)
pkdata @rtransform!(pkdata, :evid = :AMT == 0 ? 0 : 1)
@rtransform!(pkdata, :cmt = :AMT == 0 ? missing : Symbol("Central"))
@rtransform!(pkdata, :CONC = :evid == 1 ? missing : :CONC)
Then, we map the DataFrame
to a population. We can omit specifying the cmt
and evid
keyword because we used the default value of lower case :cmt
and :evid
.
= read_pumas(
pk_population
pkdata;= :CID,
id = :TIME,
time = [:CONC],
observations = :AMT,
amt = :RATE,
rate )
Population
Subjects: 20
Observations: CONC
The data can be plotted using the observations_vs_time
plot.
observations_vs_time(pk_population; axis = (title = "PK data plot",))
To emphasize individual trajectories, the sim_plot
function also works on a population.
sim_plot(pk_population; axis = (title = "PK data plot",))
3 PK Model definition
The next step is to define the PK model. Since we have IV infusion we do not need a depot. The model does not contain any significant distribution phase. With just a Central
compartment, we can use the Central1
predefined model and its associated closed form solution. This is equivalent to ADVAN1
in NONMEM.
= @model begin
inf1cmt @param begin
∈ RealDomain(lower = 0.0)
θcl ∈ RealDomain(lower = 0.0)
θvc ∈ PDiagDomain(2)
Ω ∈ RealDomain(lower = 0.0)
σ_add ∈ RealDomain(lower = 0.0)
σ_prop end
@random begin
~ MvNormal(Ω)
η end
@pre begin
= θcl * exp(η[1])
CL = θvc * exp(η[2])
Vc end
@dynamics Central1
@derived begin
:= @. Central / Vc
conc_model ~ @. Normal(conc_model, sqrt(σ_add^2 + (conc_model * σ_prop)^2))
CONC end
end
PumasModel
Parameters: θcl, θvc, Ω, σ_add, σ_prop
Random effects: η
Covariates:
Dynamical system variables: Central
Dynamical system type: Closed form
Derived: CONC
Observed: CONC
To be able to fit the model we need to specify initial parameters.
= (
initial_est_inf1cmt = 1.0,
θcl = 1.0,
θvc = Diagonal([0.09, 0.09]),
Ω = sqrt(10.0),
σ_add = sqrt(0.01),
σ_prop )
(θcl = 1.0,
θvc = 1.0,
Ω = [0.09 0.0; 0.0 0.09],
σ_add = 3.1622776601683795,
σ_prop = 0.1,)
And then we can fit the model to data.
= fit(inf1cmt, pk_population, initial_est_inf1cmt, FOCE()) inf1cmt_results
[ Info: Checking the initial parameter values.
[ Info: The initial negative log likelihood and its gradient are finite. Check passed.
Iter Function value Gradient norm
0 3.174194e+03 1.463122e+03
* time: 0.04163098335266113
1 1.700194e+03 3.899473e+02
* time: 1.6207640171051025
2 1.372117e+03 2.133637e+02
* time: 1.6440370082855225
3 1.149219e+03 9.540101e+01
* time: 1.66975998878479
4 1.063179e+03 5.172865e+01
* time: 1.693295955657959
5 1.025366e+03 2.646221e+01
* time: 1.7150590419769287
6 1.011715e+03 1.666561e+01
* time: 1.8638999462127686
7 1.007591e+03 1.182557e+01
* time: 1.8829841613769531
8 1.006663e+03 9.681515e+00
* time: 1.901414155960083
9 1.006394e+03 8.838980e+00
* time: 1.9178919792175293
10 1.006061e+03 8.916639e+00
* time: 1.9337880611419678
11 1.005287e+03 8.898501e+00
* time: 1.9500491619110107
12 1.003673e+03 1.594601e+01
* time: 1.9667601585388184
13 1.000433e+03 3.264510e+01
* time: 1.9837141036987305
14 9.939620e+02 5.680776e+01
* time: 2.000988006591797
15 9.920492e+02 8.189008e+01
* time: 2.020315170288086
16 9.853470e+02 4.792287e+01
* time: 2.0404560565948486
17 9.821580e+02 4.099167e+01
* time: 2.0563690662384033
18 9.784445e+02 4.359738e+01
* time: 2.075076103210449
19 9.753844e+02 1.715016e+01
* time: 2.0924391746520996
20 9.742523e+02 1.244736e+01
* time: 2.1068170070648193
21 9.734762e+02 1.086879e+01
* time: 2.1219639778137207
22 9.729031e+02 1.529972e+01
* time: 2.1368370056152344
23 9.714972e+02 2.051054e+01
* time: 2.152014970779419
24 9.702763e+02 1.703802e+01
* time: 2.1677279472351074
25 9.693945e+02 1.242588e+01
* time: 2.183314085006714
26 9.690627e+02 1.193813e+01
* time: 2.198141098022461
27 9.687959e+02 1.049254e+01
* time: 2.2139079570770264
28 9.683343e+02 7.801000e+00
* time: 2.229084014892578
29 9.667001e+02 1.096197e+01
* time: 2.245224952697754
30 9.660129e+02 9.792112e+00
* time: 2.2598869800567627
31 9.655534e+02 9.823330e+00
* time: 2.275177001953125
32 9.651971e+02 1.059550e+01
* time: 2.2885870933532715
33 9.636185e+02 1.011640e+01
* time: 2.305325984954834
34 9.621257e+02 7.727968e+00
* time: 2.322064161300659
35 9.603739e+02 9.857407e+00
* time: 2.339787006378174
36 9.603277e+02 1.508837e+01
* time: 2.4091410636901855
37 9.598745e+02 3.570285e-01
* time: 2.425374984741211
38 9.598694e+02 2.341098e-01
* time: 2.4400360584259033
39 9.598690e+02 9.077958e-02
* time: 2.4537999629974365
40 9.598690e+02 3.615731e-02
* time: 2.4667110443115234
41 9.598690e+02 3.631911e-03
* time: 2.479691982269287
42 9.598690e+02 3.934849e-04
* time: 2.4912450313568115
FittedPumasModel
Successful minimization: true
Likelihood approximation: FOCE
Likelihood Optimizer: BFGS
Dynamical system type: Closed form
Log-likelihood value: -959.86896
Number of subjects: 20
Number of parameters: Fixed Optimized
0 6
Observation records: Active Missing
CONC: 220 0
Total: 220 0
---------------------
Estimate
---------------------
θcl 0.024451
θvc 0.074289
Ω₁,₁ 0.072037
Ω₂,₂ 0.09384
σ_add 3.2726
σ_prop 0.10228
---------------------
4 Model diagnostics
As usual, we use the inspect
function to calculate all diagnostics.
= inspect(inf1cmt_results) inf1cmt_insp
[ Info: Calculating predictions.
[ Info: Calculating weighted residuals.
[ Info: Calculating empirical bayes.
[ Info: Evaluating individual parameters.
[ Info: Evaluating dose control parameters.
[ Info: Done.
FittedPumasModelInspection
Fitting was successful: true
Likehood approximation used for weighted residuals : Pumas.FOCE{Optim.NewtonTrustRegion{Float64}, Optim.Options{Float64, Nothing}}(Optim.NewtonTrustRegion{Float64}(1.0, 100.0, 1.4901161193847656e-8, 0.1, 0.25, 0.75, false), Optim.Options(x_abstol = 0.0, x_reltol = 0.0, f_abstol = 0.0, f_reltol = 0.0, g_abstol = 1.0e-5, g_reltol = 1.0e-8, outer_x_abstol = 0.0, outer_x_reltol = 0.0, outer_f_abstol = 0.0, outer_f_reltol = 0.0, outer_g_abstol = 1.0e-8, outer_g_reltol = 1.0e-8, f_calls_limit = 0, g_calls_limit = 0, h_calls_limit = 0, allow_f_increases = false, allow_outer_f_increases = true, successive_f_tol = 1, iterations = 1000, outer_iterations = 1000, store_trace = false, trace_simplex = false, show_trace = false, extended_trace = false, show_warnings = true, show_every = 1, time_limit = NaN, )
)
These can be saved to a file by constructing a table representation of everything from predictions to weighted residuals and empirical bayes estimes and individual coefficients (POSTHOC in NONMEM).
= DataFrame(inf1cmt_insp)
df_inspect write("inspect_file.csv", df_inspect) CSV.
"inspect_file.csv"
Besides mean predictions, it is also simple to simulate from the estimated model using the empirical bayes estimates as the values for the random effects.
sim_plot(simobs(inf1cmt, pk_population, coef(inf1cmt_results)))
For the PD model we need individual CL
and Vc
values.
= unique(df_inspect[!, [:id, :time, :CL, :Vc]], :id)
icoef_dataframe rename!(icoef_dataframe, :CL => :CLi, :Vc => :Vci)
Row | id | time | CLi | Vci |
---|---|---|---|---|
String | Float64 | Float64? | Float64? | |
1 | 1 | 0.0 | 0.0154794 | 0.0879781 |
2 | 10 | 0.0 | 0.0308652 | 0.0566826 |
3 | 11 | 0.0 | 0.028236 | 0.0814447 |
4 | 12 | 0.0 | 0.0397456 | 0.0597957 |
5 | 13 | 0.0 | 0.021964 | 0.0764398 |
6 | 14 | 0.0 | 0.0233953 | 0.115324 |
7 | 15 | 0.0 | 0.0270355 | 0.0653006 |
8 | 16 | 0.0 | 0.0253244 | 0.0579478 |
9 | 17 | 0.0 | 0.0278282 | 0.144549 |
10 | 18 | 0.0 | 0.0400327 | 0.0813417 |
11 | 19 | 0.0 | 0.0183681 | 0.0723819 |
12 | 2 | 0.0 | 0.0191042 | 0.0920158 |
13 | 20 | 0.0 | 0.0276561 | 0.0533032 |
14 | 3 | 0.0 | 0.0156637 | 0.0753704 |
15 | 4 | 0.0 | 0.0260312 | 0.0691899 |
16 | 5 | 0.0 | 0.0284244 | 0.103891 |
17 | 6 | 0.0 | 0.0245165 | 0.094743 |
18 | 7 | 0.0 | 0.0153045 | 0.0360822 |
19 | 8 | 0.0 | 0.0261443 | 0.0750091 |
20 | 9 | 0.0 | 0.0248482 | 0.0555691 |
5 Getting the data
The data file consists of data obtained from 10 individuals who were treated with 500mg dose TID (three times a day, every eight hours) for five days. The dataset exists in the PharmaDatasets
package and we load it into memory as a DataFrame
. We specify that the first column should be a String
because we want to join the individual parameters from the PK step to the PD data, and the id
column of the DataFrame
from inspect
is a String
column. We use the first(input, number_of_elements)
function to show the first 10 rows of the DataFrame
.
= CSV.read(
pddata dataset("pumas/event_data/CS3_IVINFPDEST", String),
DataFrame;= 5,
header = Dict(1 => String),
types
)rename!(pddata, :CID => :id, :TIME => :time, :AMT => :amt, :CMT => :cmt)
@rtransform!(pddata, :evid = :amt == 0 ? 0 : 1)
@rtransform!(pddata, :HIST = :evid == 1 ? missing : :HIST)
first(pddata, 10)
Row | id | time | HIST | amt | cmt | RATE | MDV | CLI | VI | evid |
---|---|---|---|---|---|---|---|---|---|---|
String | Float64 | Float64? | Int64 | Int64 | Float64 | Int64 | Float64 | Float64 | Int64 | |
1 | 1 | 0.0 | missing | 100 | 1 | 16.7 | 1 | 15.585 | 90.812 | 1 |
2 | 1 | 0.0 | missing | 1 | 2 | 0.0 | 1 | 15.585 | 90.812 | 1 |
3 | 1 | 0.0 | 13.008 | 0 | 2 | 0.0 | 0 | 15.585 | 90.812 | 0 |
4 | 1 | 0.5 | 13.808 | 0 | 2 | 0.0 | 0 | 15.585 | 90.812 | 0 |
5 | 1 | 1.0 | 8.6859 | 0 | 2 | 0.0 | 0 | 15.585 | 90.812 | 0 |
6 | 1 | 3.0 | 6.2601 | 0 | 2 | 0.0 | 0 | 15.585 | 90.812 | 0 |
7 | 1 | 5.0 | 4.0602 | 0 | 2 | 0.0 | 0 | 15.585 | 90.812 | 0 |
8 | 1 | 6.0 | 4.4985 | 0 | 2 | 0.0 | 0 | 15.585 | 90.812 | 0 |
9 | 1 | 8.0 | 3.2736 | 0 | 2 | 0.0 | 0 | 15.585 | 90.812 | 0 |
10 | 1 | 12.0 | 2.6026 | 0 | 2 | 0.0 | 0 | 15.585 | 90.812 | 0 |
The file contains 11 columns:
= outerjoin(pddata, icoef_dataframe; on = [:id, :time])
pd_dataframe sort!(pd_dataframe, [:id, :time])
Row | id | time | HIST | amt | cmt | RATE | MDV | CLI | VI | evid | CLi | Vci |
---|---|---|---|---|---|---|---|---|---|---|---|---|
String | Float64 | Float64? | Int64? | Int64? | Float64? | Int64? | Float64? | Float64? | Int64? | Float64? | Float64? | |
1 | 1 | 0.0 | missing | 100 | 1 | 16.7 | 1 | 15.585 | 90.812 | 1 | 0.0154794 | 0.0879781 |
2 | 1 | 0.0 | missing | 1 | 2 | 0.0 | 1 | 15.585 | 90.812 | 1 | 0.0154794 | 0.0879781 |
3 | 1 | 0.0 | 13.008 | 0 | 2 | 0.0 | 0 | 15.585 | 90.812 | 0 | 0.0154794 | 0.0879781 |
4 | 1 | 0.5 | 13.808 | 0 | 2 | 0.0 | 0 | 15.585 | 90.812 | 0 | missing | missing |
5 | 1 | 1.0 | 8.6859 | 0 | 2 | 0.0 | 0 | 15.585 | 90.812 | 0 | missing | missing |
6 | 1 | 3.0 | 6.2601 | 0 | 2 | 0.0 | 0 | 15.585 | 90.812 | 0 | missing | missing |
7 | 1 | 5.0 | 4.0602 | 0 | 2 | 0.0 | 0 | 15.585 | 90.812 | 0 | missing | missing |
8 | 1 | 6.0 | 4.4985 | 0 | 2 | 0.0 | 0 | 15.585 | 90.812 | 0 | missing | missing |
9 | 1 | 8.0 | 3.2736 | 0 | 2 | 0.0 | 0 | 15.585 | 90.812 | 0 | missing | missing |
10 | 1 | 12.0 | 2.6026 | 0 | 2 | 0.0 | 0 | 15.585 | 90.812 | 0 | missing | missing |
11 | 1 | 15.0 | 2.3422 | 0 | 2 | 0.0 | 0 | 15.585 | 90.812 | 0 | missing | missing |
12 | 1 | 18.0 | 3.8008 | 0 | 2 | 0.0 | 0 | 15.585 | 90.812 | 0 | missing | missing |
13 | 1 | 24.0 | 7.1397 | 0 | 2 | 0.0 | 0 | 15.585 | 90.812 | 0 | missing | missing |
⋮ | ⋮ | ⋮ | ⋮ | ⋮ | ⋮ | ⋮ | ⋮ | ⋮ | ⋮ | ⋮ | ⋮ | ⋮ |
269 | 9 | 0.0 | 34.608 | 0 | 2 | 0.0 | 0 | 24.809 | 56.453 | 0 | 0.0248482 | 0.0555691 |
270 | 9 | 0.5 | 29.57 | 0 | 2 | 0.0 | 0 | 24.809 | 56.453 | 0 | missing | missing |
271 | 9 | 1.0 | 27.68 | 0 | 2 | 0.0 | 0 | 24.809 | 56.453 | 0 | missing | missing |
272 | 9 | 3.0 | 18.397 | 0 | 2 | 0.0 | 0 | 24.809 | 56.453 | 0 | missing | missing |
273 | 9 | 5.0 | 14.908 | 0 | 2 | 0.0 | 0 | 24.809 | 56.453 | 0 | missing | missing |
274 | 9 | 6.0 | 11.669 | 0 | 2 | 0.0 | 0 | 24.809 | 56.453 | 0 | missing | missing |
275 | 9 | 8.0 | 8.5475 | 0 | 2 | 0.0 | 0 | 24.809 | 56.453 | 0 | missing | missing |
276 | 9 | 12.0 | 16.895 | 0 | 2 | 0.0 | 0 | 24.809 | 56.453 | 0 | missing | missing |
277 | 9 | 15.0 | 21.326 | 0 | 2 | 0.0 | 0 | 24.809 | 56.453 | 0 | missing | missing |
278 | 9 | 18.0 | 26.131 | 0 | 2 | 0.0 | 0 | 24.809 | 56.453 | 0 | missing | missing |
279 | 9 | 24.0 | 26.278 | 0 | 2 | 0.0 | 0 | 24.809 | 56.453 | 0 | missing | missing |
280 | 9 | 30.0 | 39.643 | 0 | 2 | 0.0 | 0 | 24.809 | 56.453 | 0 | missing | missing |
6 Converting the DataFrame to a collection of Subjects
= read_pumas(pd_dataframe; observations = [:HIST], covariates = [:CLi, :Vci]) population
Population
Subjects: 20
Covariates: CLi, Vci
Observations: HIST
7 IDR model
= @model begin
irm1 @metadata begin
= "POPULATION PK-PD MODELING"
desc = u"hr" # hour
timeu end
@param begin
∈ RealDomain(lower = 0)
tvkin ∈ RealDomain(lower = 0)
tvkout ∈ RealDomain(lower = 0)
tvic50 ∈ RealDomain(lower = 0)
tvimax ∈ PDiagDomain(3)
Ω ∈ RealDomain(lower = 0)
σ_add_pd ∈ RealDomain(lower = 0)
σ_prop_pd end
@random begin
~ MvNormal(Ω)
η end
@covariates CLi Vci
@pre begin
= tvkin * exp(η[1])
kin = tvkout * exp(η[2])
kout = kin / kout
bsl = tvic50 * exp(η[3])
ic50 = tvimax
imax = CLi
CL = Vci
Vc end
@init begin
= bsl
Response end
@dynamics begin
' = -CL / Vc * Central
Central' =
Response* (1 - imax * (Central / Vc) / (ic50 + Central / Vc)) - kout * Response
kin end
@derived begin
~ @. Normal(Response, sqrt(σ_add_pd^2 + (Response * σ_prop_pd)^2))
HIST end
end
PumasModel
Parameters: tvkin, tvkout, tvic50, tvimax, Ω, σ_add_pd, σ_prop_pd
Random effects: η
Covariates: CLi, Vci
Dynamical system variables: Central, Response
Dynamical system type: Nonlinear ODE
Derived: HIST
Observed: HIST
= (
init_θ = 5.4,
tvkin = 0.3,
tvkout = 3.9,
tvic50 = 1.0,
tvimax = Diagonal([0.2, 0.2, 0.2]),
Ω = 0.05,
σ_add_pd = 0.05,
σ_prop_pd )
(tvkin = 5.4,
tvkout = 0.3,
tvic50 = 3.9,
tvimax = 1.0,
Ω = [0.2 0.0 0.0; 0.0 0.2 0.0; 0.0 0.0 0.2],
σ_add_pd = 0.05,
σ_prop_pd = 0.05,)
= fit(
irm1_results
irm1,
population,
init_θ,FOCE();
Pumas.= (tvimax = 1.0,),
constantcoef = (show_every = 10,),
optim_options )
[ Info: Checking the initial parameter values.
[ Info: The initial negative log likelihood and its gradient are finite. Check passed.
Iter Function value Gradient norm
0 1.625453e+03 2.073489e+03
* time: 0.00017213821411132812
10 5.899604e+02 6.134653e+00
* time: 2.190984010696411
20 5.824375e+02 2.641108e+01
* time: 3.978708028793335
30 5.801204e+02 1.223991e+00
* time: 5.813814163208008
40 5.798576e+02 5.986551e-01
* time: 7.170806169509888
50 5.668947e+02 6.290151e+00
* time: 8.821391105651855
60 5.591284e+02 5.109298e+00
* time: 10.328111171722412
70 5.559638e+02 3.233225e-04
* time: 11.874957084655762
FittedPumasModel
Successful minimization: true
Likelihood approximation: FOCE
Likelihood Optimizer: BFGS
Dynamical system type: Nonlinear ODE
Solver(s):(OrdinaryDiffEq.Vern7,OrdinaryDiffEq.Rodas5P)
Log-likelihood value: -555.96385
Number of subjects: 20
Number of parameters: Fixed Optimized
1 8
Observation records: Active Missing
HIST: 240 0
Total: 240 0
------------------------
Estimate
------------------------
tvkin 5.5533
tvkout 0.27864
tvic50 30.427
tvimax 1.0
Ω₁,₁ 0.18348
Ω₂,₂ 0.067395
Ω₃,₃ 0.20683
σ_add_pd 1.1094
σ_prop_pd 0.1038
------------------------
= inspect(irm1_results)
irm1_insp goodness_of_fit(irm1_insp)
[ Info: Calculating predictions.
[ Info: Calculating weighted residuals.
[ Info: Calculating empirical bayes.
[ Info: Evaluating individual parameters.
[ Info: Evaluating dose control parameters.
[ Info: Done.
8 Conclusion
In this tutorial, we saw how to build on topics we learnt in the previous two case studies to build a sequential PKPD model. We built two different models and saw how to forward the results from the PK model to the PD model. This concludes the third of the three case studies.