using Pumas
using PharmaDatasets
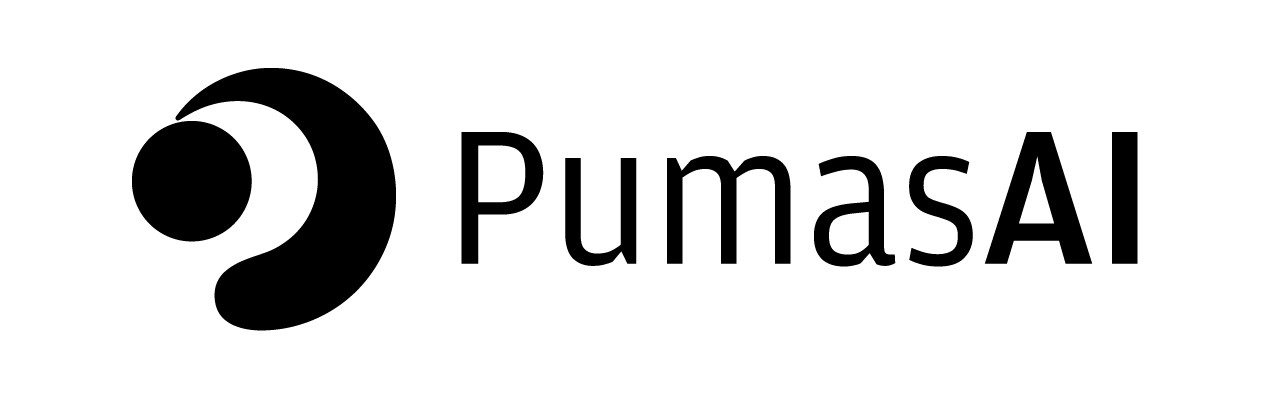
Covariate Selection Methods - Introduction
In pharmacometric workflows, we often have competing models to select from. In this tutorial we will review selection criteria and automated procedures to select the best model out of a set of competing candidate models.
First, we’ll review how to measure model fit, then we’ll cover model selection algorithms.
1 Model Fit Measures
Traditionally in Statistics, model comparison has been done based on a theoretical divergence metric that originates from information theory’s entropy:
\[H = - \operatorname{E}\log(p) = -\sum_i p_i \log(p_i)\]
where \(p_i\) is the probability of occurrence of the \(i\)-th possible value.
We use the \(\log\) scale because it transforms a product of probabilities into a sum, which is both numerically faster and numerically more stable due to the robustness against floating point underflow.
Entropy was the inspiration behind Akaike’s Information Criterion (AIC) (Akaike, 1973):
\[\operatorname{AIC} = -2\log{\hat{\mathcal{L}}} + 2k\]
where \(\hat{\mathcal{L}}\) is the estimated value of the likelihood for a given model and data, and \(k\) is the number of parameters in the model. Generally the likelihood is estimated by maximizing the likelihood function, thus the name maximum likelihood estimation (MLE). The likelihood describes how well the model fits the data, and in certain conditions, can be treated similarly to a probability: higher values means higher plausibility. Hence, models with higher likelihood values demonstrate better fits to the data. Since we are multiplying by a negative value, this means that lower values are preferred.
The \(-2\) was proposed in Akaike’s 1973 original paper to simplify some calculations involving \(\chi^2\) distributions and was kept around since then.
AIC was devised to “punish” model complexity, i.e models that have more parameters to fit to the data. This is why we add \(2\) to the loglikelihood value for every parameter that the model has. Due to the preference of lower AIC values this penalizes models by the number of parameters, while also making it possible to compare models with different complexities.
Building from the AIC, the Bayesian Information Criterion (BIC) (Schwarz, 1978) uses the same idea, but the penalty term is different:
\[\operatorname{BIC} = -2\log{\hat{\mathcal{L}}} + k\log(n)\]
where \(\hat{\mathcal{L}}\) is the estimated value of the likelihood for a given model and data, \(k\) is the model’s number of parameters, and \(n\) is the number of observations. It is called Bayesian because it uses a “Bayesian” argument to derive the punishment term \(k\log(n)\) in the original 1975 paper.
1.1 Example in Pumas
Let’s go over an example of model fit measures in Pumas.
First, let’s import the following packages:
We are going to use the po_sad_1
dataset from PharmaDatasets
:
= dataset("po_sad_1")
df first(df, 5)
Row | id | time | dv | amt | evid | cmt | rate | age | wt | doselevel | isPM | isfed | sex | route |
---|---|---|---|---|---|---|---|---|---|---|---|---|---|---|
Int64 | Float64 | Float64? | Float64? | Int64 | Int64? | Float64 | Int64 | Int64 | Int64 | String3 | String3 | String7 | String3 | |
1 | 1 | 0.0 | missing | 30.0 | 1 | 1 | 0.0 | 51 | 74 | 30 | no | yes | male | ev |
2 | 1 | 0.25 | 35.7636 | missing | 0 | missing | 0.0 | 51 | 74 | 30 | no | yes | male | ev |
3 | 1 | 0.5 | 71.9551 | missing | 0 | missing | 0.0 | 51 | 74 | 30 | no | yes | male | ev |
4 | 1 | 0.75 | 97.3356 | missing | 0 | missing | 0.0 | 51 | 74 | 30 | no | yes | male | ev |
5 | 1 | 1.0 | 128.919 | missing | 0 | missing | 0.0 | 51 | 74 | 30 | no | yes | male | ev |
This is an oral dosing (route = "ev"
) NMTRAN-formatted dataset. It has 18 subjects, each with 1 dosing event (evid = 1
) and 18 measurement events (evid = 0
); and the following covariates:
age
: age in years (continuous)wt
: weight in kg (continuous)doselevel
: dosing amount, either30
,60
or90
milligrams (categorical)isPM
: subject is a poor metabolizer (binary)isfed
: subject is fed (binary)sex
: subject sex (binary)
Let’s parse df
into a Population
with read_pumas
:
=
population read_pumas(df; observations = [:dv], covariates = [:wt, :isPM, :isfed], route = :route)
Population
Subjects: 18
Covariates: wt, isPM, isfed
Observations: dv
Let’s create a 2-compartment oral absorption base model with no covariate effects:
= @model begin
base_model @metadata begin
= "base model"
desc = u"hr"
timeu end
@param begin
"""
Clearance (L/hr)
"""
∈ RealDomain(; lower = 0)
tvcl """
Central Volume (L)
"""
∈ RealDomain(; lower = 0)
tvvc """
Peripheral Volume (L)
"""
∈ RealDomain(; lower = 0)
tvvp """
Distributional Clearance (L/hr)
"""
∈ RealDomain(; lower = 0)
tvq """
Absorption rate constant (1/h)
"""
∈ RealDomain(; lower = 0)
tvka """
- ΩCL
- ΩVc
- ΩKa
- ΩVp
- ΩQ
"""
∈ PDiagDomain(5)
Ω """
Proportional RUV (SD scale)
"""
∈ RealDomain(; lower = 0)
σₚ end
@random begin
~ MvNormal(Ω)
η end
@pre begin
= tvcl * exp(η[1])
CL = tvvc * exp(η[2])
Vc = tvka * exp(η[3])
Ka = tvq * exp(η[4])
Q = tvvp * exp(η[5])
Vp end
@dynamics Depots1Central1Periph1
@derived begin
:= @. 1_000 * (Central / Vc)
cp """
Drug Concentration (ng/mL)
"""
~ @. Normal(cp, cp * σₚ)
dv end
end
PumasModel
Parameters: tvcl, tvvc, tvvp, tvq, tvka, Ω, σₚ
Random effects: η
Covariates:
Dynamical system variables: Depot, Central, Peripheral
Dynamical system type: Closed form
Derived: dv
Observed: dv
Let’s go over the model.
In the @metadata
block we are adding a model description and adding information regarding the time units (hours).
Next, we define the model’s parameters in @param
while also prepending them with a string that serves as an annotation for the parameter description. This is helpful for post-processing, since Pumas can use the description instead of the parameter name in tables and figures.
Our η
s are defined in the @random
block and are sampled from a multivariate normal distribution with mean 0
and a positive-diagonal covariance matrix Ω
. We have 5 η
s, one for each PK typical value (also known as θ
s).
We proceed by defining the individual PK parameters in the @pre
block. Each typical value is incremented by the subject’s η
s in a non-linear exponential transformation. This is done to enforce that all individual PK parameters are constrained to being positive. This also has a side effect that the individual PK parameters will be log-normally distributed.
We use the aliased short notation Depots1Central1Periph1
for the ODE system in the @dynamics
. This is equivalent to having the following equations:
' = -Ka * Depot
Depot' = Ka * Depot - (CL + Q) / Vc * Central + Q / Vp * Peripheral
Central' = Q / Vc * Central - Q / Vp * Peripheral Peripheral
Note that, in order for Depots1Central1Periph1
work correctly, we need to define Ka
, CL
, Q
, Vc
, and Vp
in the @pre
block.
Finally, in the @derived
block we define our error model (or likelihood for the statistically-minded). Here we are using a proportional error model with the Gaussian/normal likelihood. Note that Normal
is parameterized with mean and standard deviation, not with variance. That’s why we name our proportional error parameter as σₚ
and not σ²ₚ
.
Let’s now define a initial set of parameter estimates to fit our model:
= (;
iparams = 0.4,
tvka = 4.0,
tvcl = 70.0,
tvvc = 4.0,
tvq = 50.0,
tvvp = Diagonal(fill(0.04, 5)),
Ω = 0.1,
σₚ )
(tvka = 0.4,
tvcl = 4.0,
tvvc = 70.0,
tvq = 4.0,
tvvp = 50.0,
Ω = [0.04 0.0 … 0.0 0.0; 0.0 0.04 … 0.0 0.0; … ; 0.0 0.0 … 0.04 0.0; 0.0 0.0 … 0.0 0.04],
σₚ = 0.1,)
We call the fit
function to estimate the parameters of the model:
= fit(base_model, population, iparams, FOCE()) base_fit
[ Info: Checking the initial parameter values.
[ Info: The initial negative log likelihood and its gradient are finite. Check passed.
Iter Function value Gradient norm
0 1.630402e+03 2.604358e+02
* time: 0.025423049926757812
1 1.499510e+03 9.365700e+01
* time: 1.0270721912384033
2 1.447619e+03 4.714464e+01
* time: 1.0758440494537354
3 1.427906e+03 4.439232e+01
* time: 1.124704122543335
4 1.414326e+03 2.726109e+01
* time: 1.18174409866333
5 1.387798e+03 1.159019e+01
* time: 1.238448143005371
6 1.382364e+03 7.060796e+00
* time: 1.319965124130249
7 1.380839e+03 4.839103e+00
* time: 1.3671200275421143
8 1.380281e+03 4.075615e+00
* time: 1.4173572063446045
9 1.379767e+03 3.303901e+00
* time: 1.4732990264892578
10 1.379390e+03 2.856359e+00
* time: 1.5430481433868408
11 1.379193e+03 2.650736e+00
* time: 1.587245225906372
12 1.379036e+03 2.523349e+00
* time: 1.6318750381469727
13 1.378830e+03 2.638648e+00
* time: 1.6844401359558105
14 1.378593e+03 3.463990e+00
* time: 1.7388741970062256
15 1.378335e+03 3.471127e+00
* time: 1.8080940246582031
16 1.378143e+03 2.756670e+00
* time: 1.8539061546325684
17 1.378019e+03 2.541343e+00
* time: 1.900785207748413
18 1.377888e+03 2.163251e+00
* time: 1.9566550254821777
19 1.377754e+03 2.571076e+00
* time: 2.030708074569702
20 1.377620e+03 3.370764e+00
* time: 2.0783400535583496
21 1.377413e+03 3.938291e+00
* time: 2.1250741481781006
22 1.377094e+03 4.458016e+00
* time: 2.176117181777954
23 1.376674e+03 5.713348e+00
* time: 2.2328250408172607
24 1.375946e+03 5.417530e+00
* time: 2.3080570697784424
25 1.375343e+03 5.862876e+00
* time: 2.3591840267181396
26 1.374689e+03 5.717165e+00
* time: 2.4101030826568604
27 1.374056e+03 4.400490e+00
* time: 2.469898223876953
28 1.373510e+03 2.191437e+00
* time: 2.5497450828552246
29 1.373277e+03 1.203587e+00
* time: 2.6048672199249268
30 1.373233e+03 1.157761e+00
* time: 2.657543182373047
31 1.373218e+03 8.770728e-01
* time: 2.713285207748413
32 1.373204e+03 8.021952e-01
* time: 2.772830009460449
33 1.373190e+03 6.613857e-01
* time: 2.845196008682251
34 1.373183e+03 7.602394e-01
* time: 2.894728183746338
35 1.373173e+03 8.552154e-01
* time: 2.9496982097625732
36 1.373162e+03 6.961928e-01
* time: 3.0107290744781494
37 1.373152e+03 3.162546e-01
* time: 3.083862066268921
38 1.373148e+03 1.747381e-01
* time: 3.133486032485962
39 1.373147e+03 1.258699e-01
* time: 3.181230068206787
40 1.373147e+03 1.074908e-01
* time: 3.2408580780029297
41 1.373147e+03 6.799619e-02
* time: 3.2999460697174072
42 1.373147e+03 1.819329e-02
* time: 3.3645660877227783
43 1.373147e+03 1.338880e-02
* time: 3.4077680110931396
44 1.373147e+03 1.370144e-02
* time: 3.4565320014953613
45 1.373147e+03 1.315666e-02
* time: 3.510148048400879
46 1.373147e+03 1.065953e-02
* time: 3.580620050430298
47 1.373147e+03 1.069775e-02
* time: 3.6245391368865967
48 1.373147e+03 6.234846e-03
* time: 3.6658670902252197
49 1.373147e+03 6.234846e-03
* time: 3.745281219482422
50 1.373147e+03 6.234846e-03
* time: 3.86984920501709
FittedPumasModel
Successful minimization: true
Likelihood approximation: FOCE
Likelihood Optimizer: BFGS
Dynamical system type: Closed form
Log-likelihood value: -1373.1468
Number of subjects: 18
Number of parameters: Fixed Optimized
0 11
Observation records: Active Missing
dv: 270 0
Total: 270 0
-------------------
Estimate
-------------------
tvcl 2.8344
tvvc 77.801
tvvp 48.754
tvq 3.9789
tvka 1.028
Ω₁,₁ 0.2638
Ω₂,₂ 0.2288
Ω₃,₃ 0.40047
Ω₄,₄ 0.37968
Ω₅,₅ 0.21495
σₚ 0.097805
-------------------
Now we are ready to showcase model fit measures. All of these functions should take a result from fit
and output a real number.
Let’s start with aic
and bic
which are included in Pumas:
aic(base_fit)
2768.2935804173985
bic(base_fit)
2807.876221966381
We are also free to create our own functions if we want to use something different than aic
or bic
.
Here’s an example of a function that takes a fitted Pumas model, m
, and outputs the -2LL (minus 2 times log-likelihood) without the constant. This is a model fit measure commonly used by NONMEM users and is is known as OFV: Objective Function Value. Hence, we will name the function ofv
:
ofv(m) = (-2 * loglikelihood(m)) - (nobs(m) * log(2π))
ofv (generic function with 1 method)
We can use it on our base_fit
model fit
result:
ofv(base_fit)
2250.0667724868754
2 Likelihood Ratio Tests
A likelihood-ratio test (LRT) is a statistical hypothesis test used in the field of statistics and probability theory to compare two statistical models and determine which one provides a better fit to a given set of observed data. It is particularly useful in the context of maximum likelihood estimation (MLE) and is commonly used for hypothesis testing in parametric statistical modeling.
The basic idea behind the likelihood ratio test is to compare the likelihoods of two competing models:
Null Hypothesis (\(H_0\)): This is the model that you want to test against. It represents a specific set of parameter values or restrictions on the model.
Alternative Hypothesis (\(H_a\)): This is the alternative model, often a more complex one or the one you want to support.
The test statistic is calculated as the ratio of the likelihood under the alternative model (\(H_a\)) to the likelihood under the null model (\(H_0\)). Mathematically, it can be expressed as:
\[\operatorname{LRT} = - 2 \log \left( \frac{\mathcal{L}(H_0)}{\mathcal{L}(H_a)} \right)\]
where:
- \(\operatorname{LRT}\): likelihood ratio test statistic
- \(\mathcal{L}(H_0)\): likelihood under \(H_0\), the likelihood of the data under the null hypothesis
- \(\mathcal{L}(H_a)\): likelihood under \(H_a\), the likelihood of the data under the alternative hypothesis
The LRT statistic follows a \(\chi^2\) (chi-squared) distribution with degrees of freedom equal to the difference in the number of parameters between the two models (i.e., the degrees of freedom is the number of additional parameters in the alternative model). In practice, you compare the LRT statistic to \(\chi^2\) distribution to determine whether the alternative model is a significantly better fit to the data than the null model.
The key idea is that if the p-value derived from the LRT statistic is lower than your desired \(\alpha\) (the type-1 error rate, commonly set to \(0.05\)), you would reject the null hypothesis in favor of the alternative hypothesis, indicating that the alternative model provides a better fit to the data.
The likelihood-ratio test requires that the models be nested, i.e. the more complex model can be transformed into the simpler model by imposing constraints on the former’s parameters.
This is generally the case when performing LRT in a covariate selection context. However, be mindful of not violating this assumption when performing LRT.
2.1 Example in Pumas
Pumas provides us with the lrtest
function to perform LRT. It takes 2 positional arguments as competing models:
- Model under \(H_0\) (i.e. the model with less parameters)
- Model under \(H_a\) (i.e. the model with more parameters)
Let’s define a covariate model that takes wt
into consideration for all the clearance and volume PK parameters:
= @model begin
covariate_model @metadata begin
= "covariate model that uses weight covariate information"
desc = u"hr"
timeu end
@param begin
"""
Clearance (L/hr)
"""
∈ RealDomain(; lower = 0)
tvcl """
Central Volume (L)
"""
∈ RealDomain(; lower = 0)
tvvc """
Peripheral Volume (L)
"""
∈ RealDomain(; lower = 0)
tvvp """
Distributional Clearance (L/hr)
"""
∈ RealDomain(; lower = 0)
tvq """
Absorption rate constant (h-1)
"""
∈ RealDomain(; lower = 0)
tvka """
Power exponent on weight for Clearance # new
"""
∈ RealDomain() # new
dwtcl """
Power exponent on weight for Distributional Clearance # new
"""
∈ RealDomain() # new
dwtq """
- ΩCL
- ΩVc
- ΩKa
- ΩVp
- ΩQ
"""
∈ PDiagDomain(5)
Ω """
Proportional RUV (SD scale)
"""
∈ RealDomain(; lower = 0)
σₚ end
@random begin
~ MvNormal(Ω)
η end
@covariates begin
"""
Weight (kg) # new
"""
# new
wt end
@pre begin
= tvcl * exp(η[1]) * (wt / 70)^dwtcl # new
CL = tvvc * exp(η[2]) * (wt / 70) # new
Vc = tvka * exp(η[3])
Ka = tvq * exp(η[4]) * (wt / 70)^dwtq # new
Q = tvvp * exp(η[5]) * (wt / 70) # new
Vp end
@dynamics Depots1Central1Periph1
@derived begin
:= @. 1000 * (Central / Vc)
cp """
Drug Concentration (ng/mL)
"""
~ @. Normal(cp, cp * σₚ)
dv end
end
PumasModel
Parameters: tvcl, tvvc, tvvp, tvq, tvka, dwtcl, dwtq, Ω, σₚ
Random effects: η
Covariates: wt
Dynamical system variables: Depot, Central, Peripheral
Dynamical system type: Closed form
Derived: dv
Observed: dv
This is almost the same model as before. However, we are adding a few tweaks (commented with # new
):
wt
in the new@covariates
block- allometric scaling based on
wt
for the individual PK parametersCL
,Q
,Vc
andVp
- new parameters in
@param
for the exponent of the power function ofwt
on both individual clearance PK parametersCL
andQ
Since covariate_model
has two new parameters in the @param
block, we need to add them to the initial set of parameter estimates. We can do this by creating a new NamedTuple
that builts upon the last one iparams
, while also adding initial values for dwtcl
and dwtq
:
= (; iparams..., dwtcl = 0.75, dwtq = 0.75) iparams_covariate
(tvka = 0.4,
tvcl = 4.0,
tvvc = 70.0,
tvq = 4.0,
tvvp = 50.0,
Ω = [0.04 0.0 … 0.0 0.0; 0.0 0.04 … 0.0 0.0; … ; 0.0 0.0 … 0.04 0.0; 0.0 0.0 … 0.0 0.04],
σₚ = 0.1,
dwtcl = 0.75,
dwtq = 0.75,)
We are using Julia’s splatting ...
operator to expand inline the iparams
NamedTuple
.
Now we fit
our covariate_model
:
= fit(covariate_model, population, iparams_covariate, FOCE()) covariate_fit
[ Info: Checking the initial parameter values.
[ Info: The initial negative log likelihood and its gradient are finite. Check passed.
Iter Function value Gradient norm
0 1.555051e+03 2.584685e+02
* time: 7.295608520507812e-5
1 1.436886e+03 9.959639e+01
* time: 0.10085201263427734
2 1.383250e+03 3.318037e+01
* time: 0.14573097229003906
3 1.372961e+03 2.525341e+01
* time: 0.19175004959106445
4 1.365242e+03 2.081002e+01
* time: 0.24511408805847168
5 1.350200e+03 1.667386e+01
* time: 0.3036689758300781
6 1.346374e+03 9.195785e+00
* time: 0.38658595085144043
7 1.344738e+03 8.614309e+00
* time: 0.42954206466674805
8 1.343902e+03 4.950745e+00
* time: 0.47176694869995117
9 1.343662e+03 1.478699e+00
* time: 0.5242140293121338
10 1.343626e+03 9.575005e-01
* time: 0.576915979385376
11 1.343609e+03 8.509968e-01
* time: 0.64438796043396
12 1.343589e+03 7.964671e-01
* time: 0.689608097076416
13 1.343567e+03 8.202459e-01
* time: 0.7319040298461914
14 1.343550e+03 8.133359e-01
* time: 0.7808630466461182
15 1.343542e+03 6.865506e-01
* time: 0.8324038982391357
16 1.343538e+03 3.869567e-01
* time: 0.8965721130371094
17 1.343534e+03 2.805019e-01
* time: 0.9408879280090332
18 1.343531e+03 3.271442e-01
* time: 0.9857409000396729
19 1.343529e+03 4.584302e-01
* time: 1.036750078201294
20 1.343527e+03 3.951940e-01
* time: 1.0852720737457275
21 1.343525e+03 1.928385e-01
* time: 1.1492249965667725
22 1.343524e+03 1.958575e-01
* time: 1.193044900894165
23 1.343523e+03 2.008844e-01
* time: 1.236711025238037
24 1.343522e+03 1.636364e-01
* time: 1.286181926727295
25 1.343522e+03 1.041929e-01
* time: 1.3369710445404053
26 1.343521e+03 7.417497e-02
* time: 1.397395133972168
27 1.343521e+03 7.297961e-02
* time: 1.4364778995513916
28 1.343521e+03 8.109591e-02
* time: 1.4766991138458252
29 1.343520e+03 7.067080e-02
* time: 1.5255451202392578
30 1.343520e+03 5.088025e-02
* time: 1.5751359462738037
31 1.343520e+03 4.980085e-02
* time: 1.6603429317474365
32 1.343520e+03 4.778940e-02
* time: 1.7028980255126953
33 1.343520e+03 5.667067e-02
* time: 1.7421760559082031
34 1.343520e+03 5.825591e-02
* time: 1.7918260097503662
35 1.343519e+03 5.354660e-02
* time: 1.841494083404541
36 1.343519e+03 5.300792e-02
* time: 1.906177043914795
37 1.343519e+03 4.011720e-02
* time: 1.9474430084228516
38 1.343519e+03 3.606197e-02
* time: 1.9859039783477783
39 1.343519e+03 3.546034e-02
* time: 2.0306849479675293
40 1.343519e+03 3.525307e-02
* time: 2.0770959854125977
41 1.343519e+03 3.468091e-02
* time: 2.140177011489868
42 1.343519e+03 3.313732e-02
* time: 2.1813790798187256
43 1.343518e+03 4.524162e-02
* time: 2.221630096435547
44 1.343518e+03 5.769309e-02
* time: 2.2672500610351562
45 1.343518e+03 5.716613e-02
* time: 2.313401937484741
46 1.343517e+03 4.600797e-02
* time: 2.374666929244995
47 1.343517e+03 3.221948e-02
* time: 2.415477991104126
48 1.343517e+03 2.610758e-02
* time: 2.454970121383667
49 1.343517e+03 2.120270e-02
* time: 2.500852108001709
50 1.343517e+03 1.887916e-02
* time: 2.5493340492248535
51 1.343517e+03 1.229271e-02
* time: 2.609714984893799
52 1.343517e+03 4.778802e-03
* time: 2.64882493019104
53 1.343517e+03 2.158460e-03
* time: 2.6882970333099365
54 1.343517e+03 2.158460e-03
* time: 2.7610270977020264
55 1.343517e+03 2.158460e-03
* time: 2.8647639751434326
FittedPumasModel
Successful minimization: true
Likelihood approximation: FOCE
Likelihood Optimizer: BFGS
Dynamical system type: Closed form
Log-likelihood value: -1343.5173
Number of subjects: 18
Number of parameters: Fixed Optimized
0 13
Observation records: Active Missing
dv: 270 0
Total: 270 0
--------------------
Estimate
--------------------
tvcl 2.7287
tvvc 70.681
tvvp 47.396
tvq 4.0573
tvka 0.98725
dwtcl 0.58351
dwtq 1.176
Ω₁,₁ 0.21435
Ω₂,₂ 0.050415
Ω₃,₃ 0.42468
Ω₄,₄ 0.040356
Ω₅,₅ 0.045987
σₚ 0.097904
--------------------
Now we are ready to perform LRT with lrtest
:
= lrtest(base_fit, covariate_fit) mytest
Statistic: 59.3
Degrees of freedom: 2
P-value: 0.0
The degrees of freedom of the underlying \(\chi^2\) distribution is \(2\), i.e. we have two additional parameters in the model under \(H_a\); and the test statistic is \(59.3\).
The \(p\)-value corresponding for the test statistic and degree of freedom is very close to \(0\). It prints as 0.0
, but we can access the value with the pvalue
function:
pvalue(mytest)
1.3554737256701043e-13
This indicates strong evidence against the base_model
(i.e. model under \(H_0\)) and in favor of the covariate_model
(i.e. model under \(H_a\)).
3 Model Selection Algorithms
There are several model selection techniques that take into account covariate selection. In the statistical literature, the reader can check Thayer (1990), and for the pharmacometric context, the reader can check Hutmacher & Kowalski (2015) and Jonsson & Karlsson (1998).
Pumas currently only implements the Stepwise Covariate Model (SCM). SCM, also known as stepwise procedures, is a model building strategy that is used to identify the best covariate model for a given dataset by a series of iterations (Hutmacher & Kowalski, 2015). Broadly, there are two main types of SCM:
- Forward Selection (FS)
- Backward Elimination (BE)
We will be covering these in detail in a new set of tutorials, please check tutorials.pumas.ai.
4 References
Akaike, H. (1973). Information theory and the extension of the maximum likelihood principle. Proceedings of the Second International Symposium on Information Theory.
Hutmacher, M. M., & Kowalski, K. G. (2015). Covariate selection in pharmacometric analyses: a review of methods. British journal of clinical pharmacology, 79(1), 132–147. https://doi.org/10.1111/bcp.12451
Jonsson, E. N., & Karlsson, M. O. (1998). Automated covariate model building within NONMEM. Pharmaceutical research, 15(9), 1463–1468. https://doi.org/10.1023/a:1011970125687
Schwarz, Gideon E. (1978). Estimating the dimension of a model. Annals of Statistics, 6 (2): 461–464, doi:10.1214/aos/1176344136.
Thayer, J. D. (1990). Implementing Variable Selection Techniques in Regression. ERIC.