using Pumas
using Distributions
using DataFrames
using DataFramesMeta
using CairoMakie
using AlgebraOfGraphics
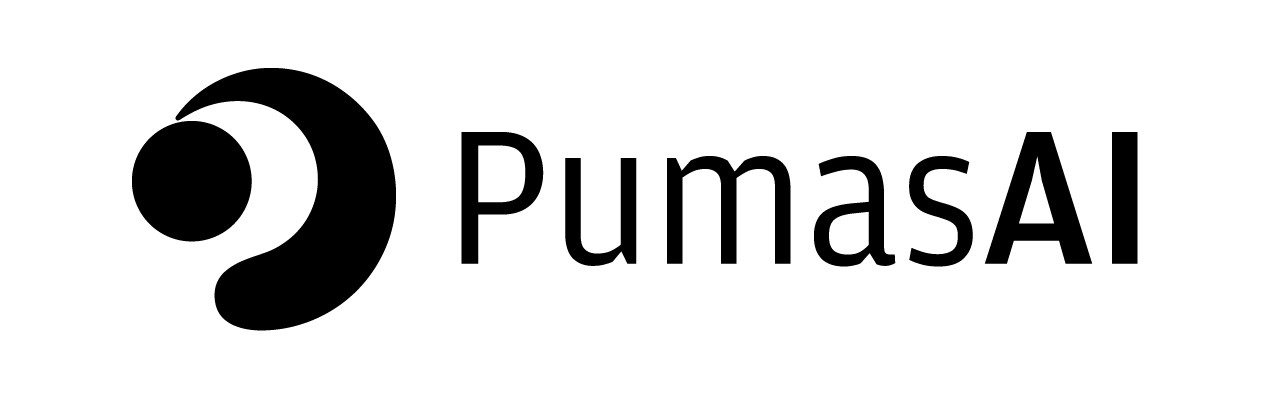
Ordinal Regression
Ordinal regression is a regression model for discrete data, and more specifically, when the dependent variable values have a “natural order”.
For example, opinion pools with their ubiquously disagree-agree range of plausible values, or a patient pain perception.
There are several resources available for a deep dive into ordinal regression. Specifically, we recommend:
- Richard McElreath - Statistical Rethinking, 2nd Edition, Section 12.3.
- Agresti, A. (2014). Categorical Data Analysis, Section 8.2.
- Bürkner, P.-C., & Vuorre, M. (2019). Ordinal Regression Models in Psychology: A Tutorial. Advances in Methods and Practices in Psychological Science, 77–101. 10.1177/2515245918823199.
1 Why not just use Linear Regression?
The main reason for not using plain linear regression with ordinal outcomes (dependent variable) is that the response categories of an ordinal variable may not be equidistant. This is an assumption in linear regression (and in almost all models that uses metric dependent variables): the distance between, for example, 2 and 3 is not the same distance between 1 and 2. This assumption can be violated in an ordinal regression.
2 How to deal with Ordered Discrete Dependent Variable?
So, how we deal with ordered discrete responses in our dependent variable? This is similar with the previous logistic regression approach. We have to do a non-linear transformation of the dependent variable.
2.1 Cumulative Distribution Function (CDF)
In the case of ordinal regression, we need to first transform the dependent variable into a cumulative scale. We need to calculate the cumulative distribution function (CDF) of our dependent variable:
\[P(Y \leq y) = \sum^y_{i=y_{\text{min}}} P(Y = i)\]
The CDF is a monotonic increasing function that depicts the probability of our random variable \(Y\) taking values less than a certain value. In our case, the discrete ordinal case, these values can be represented as positive integers ranging from 1 to the length of possible values. For instance, a 6-categorical ordered response variable will have 6 possible values, and all their CDFs will lie between 0 and 1. Furthermore, their sum will be 1; since it represents the total probability of the variable taking any of the possible values, i.e. 100%.
2.2 Log-cumulative-odds
That is still not enough, we need to apply the logit function to the CDF:
\[\mathrm{logit}(x) = \mathrm{logistic}^{-1}(x) = \ln\left(\frac{x}{1 -x}\right)\]
where \(\ln\) is the natural logarithm function.
The logit is the inverse of the logistic transformation, it takes as a input any number between 0 and 1 (where a probability is the perfect candidate) and outputs a real number, which we call the log-odds.
Odds provide a measure of the likelihood of a particular outcome. They are calculated as the ratio of the number of events that produce that outcome to the number that do not. To convert probability \(p\) to \(\mathrm{odds}\) you can use the formula:
\[\mathrm{odds}(p) = \frac{p}{1-p}\]
The higher the odds, the higher the probability.
Since we are taking the log-odds of the CDF, we can call this complete transformation as log-odds of the CDF, or log-cumulative-odds.
2.3 \(K-1\) Intercepts
Now, the next question is: what do we do with the log-cumulative-odds?
We need the log-cumulative-odds because it allows us to construct different intercepts for the possible values our ordinal dependent variable. We create an unique intercept for each possible outcome \(k \in K\).
Notice that the highest probable value of \(Y\) will always have a log-cumulative-odds of \(\infty\), since for \(p=1\):
\[\ln \frac{p}{1-p} = \ln \frac{1}{1-1} = \ln 0 = \infty\]
Thus, we only need \(K-1\) intercepts for a \(K\) possible dependent variables’ response values.
These are known as cut points.
Each intercept implies a CDF for each value \(K\). This allows us to violate the equidistant assumption absent in most ordinal variables.
Each intercept implies a log-cumulative-odds for each \(k \in K\). We also need to undo the cumulative nature of the \(K-1\) intercepts. We can accomplish this by first converting a log-cumulative-odds back to a cumulative probability. This is done by undoing the logit transformation and applying the logistic function:
\[\mathrm{logit}^{-1}(x) = \mathrm{logistic}(x) = \frac{1}{1 + e^{-x}}\]
Then, finally, we can remove the cumulative from “cumulative probability” by subtraction of each of the \(k\) cut points by their previous \(k-1\) cut point:
\[P(Y=k) = P(Y \leq k) - P(Y \leq k-1)\]
where \(Y\) is the dependent variable and \(k \in K\) are the cut points for each intercept.
2.3.1 Example with Synthetic Data
Let us show an example with some synthetic data.
First, we’ll load some packages:
Here we have a discrete variable x
with 6 possible ordered values as response. The values range from 1 to 6 having probability, respectively: 10%, 15%, 33%, 25%, 10%, and 7%; represented with the probs
vector. The underlying distribution is represented by a Categorical
distribution from Distributions.jl
, which takes a vector of probabilities as parameters (probs
).
For each value we are calculating:
- Probability Mass Function with the
pdf
function - Cumulative Distribution Function with the
cdf
function - Log-cumulative-odds with the
logit
transformation of the CDF
In the plot below there are 3 subplots:
- Upper corner: histogram of
x
- Left lower corner: CDF of
x
- Right lower corner: log-cumulative-odds of
x
= [0.10, 0.15, 0.33, 0.25, 0.10, 0.07]
probs = Categorical(probs)
dist = 1:length(probs)
x = pdf.(dist, x)
x_pmf = cdf.(dist, x)
x_cdf = logit.(x_cdf)
x_logodds_cdf = DataFrame(; x, x_pmf, x_cdf, x_logodds_cdf)
df = ["CDF", "Log-cumulative-odds"]
labels = Figure()
fig = data(df) * mapping(:x, :x_pmf) * visual(BarPlot)
plt1 =
plt2 data(df) *
mapping(:x, [:x_cdf, :x_logodds_cdf]; col = dims(1) => renamer(labels)) *
visual(ScatterLines)
= (; xticks = 1:6)
axis draw!(fig[1, 2:3], plt1; axis)
draw!(fig[2, 1:4], plt2; axis, facet = (; linkyaxes = :none))
fig
We are using the convenient logit
function from StatsFuns.jl
package. It is safer and more efficient than a simple user-defined function.
As we can see, we have \(K-1\) (in our case \(6-1=5\)) intercept values in log-cumulative-odds. You can carly see that these values they violate the equidistant assumption for metric response values. The spacing between the cut points are not the same, they vary.
We generally assign \(\alpha\) to intercept in statistical models. Since we have \(K-1\) intercepts, we need a vector of intercepts. Thus, our single parameter \(\alpha\) turns into a vector parameter with a bold \(\boldsymbol{\alpha}\) to denote it is a vector.
3 Adding Coefficients \(\boldsymbol{\beta}\)
Ok, the \(K-1\) intercepts \(\boldsymbol{\alpha}\) are done. Now let’s add coefficients to act as covariate effects in our ordinal regression model.
We transformed everything into log-odds scale so that we can add effects (coefficients multiplying a covariate) or basal rates (intercepts) together. For each \(k \in K-1\), we calculate:
\[\phi_k = \alpha_k + \beta_i x_i\]
where \(\alpha_k\) is the log-cumulative-odds for the \(k \in K-1\) intercepts, \(\beta_i\) is the coefficient for the \(i\)th covariate \(x\). Finally, \(\phi_k\) represents the linear predictor for the \(k\)th intercept.
Observe that the coefficient \(\beta\) is being added to a log-cumulative-odds, such that, it will be expressed in a log-cumulative-odds also.
We can express it in matrix form:
\[\boldsymbol{\phi} = \boldsymbol{\alpha} + \mathbf{X} \cdot \boldsymbol{\beta}\]
where \(\boldsymbol{\phi}\), \(\boldsymbol{\alpha}\) and \(\boldsymbol{\beta}\) are vectors and \(\mathbf{X}\) is the data matrix where each row is an observation and each column a covariate.
This still obeys the ordered constraint on the dependent variable possible values.
3.0.1 How to Interpret Coefficient \(\boldsymbol{\beta}\)?
Now, suppose we have found our ideal value for our \(\boldsymbol{\beta}\). How we would interpret our \(\boldsymbol{\beta}\) estimated values?
First, to recap, \(\boldsymbol{\beta}\) measures the strength of association between the covariate \(\mathbf{x}\) and dependent variable \(\mathbf{y}\). But, \(\boldsymbol{\beta}\) is nested inside a non-linear transformation called logistic function:
\[\mathrm{logistic}(\boldsymbol{\beta}) = \frac{1}{1 + e^{-\boldsymbol{\beta}}}\]
So, our first step is to undo the logistic function. There is a function that is called logit function that is the inverse of the logistic function:
\[\mathrm{logistic}^{-1}(x) = \mathrm{logit}(x) = \ln\left(\frac{x}{1 -x}\right)\]
where \(\ln\) is the natural logarithm function.
If we analyze closely the logit function we can find that inside the \(\ln\) there is a disguised odds in the \(\frac{x}{1 -x}\). Hence, our \(\boldsymbol{\beta}\) can be interpreted as the logarithm of the odds, or in short form: the log-odds.
Odds provide a measure of the likelihood of a particular outcome. They are calculated as the ratio of the number of events that produce that outcome to the number that do not. To convert probability \(p\) to \(\mathrm{odds}\) you can use the formula:
\[\mathrm{odds}(p) = \frac{p}{1-p}\]
The higher the odds, the higher the probability.
Since odds take values between \((0, \infty)\), and 1 is interpreted as neutral odds (like probability 0.5); the log-odds have a nice intuition that any negative value, that is the \(\ln\) of something between \((0, 1)\), enhances the probability of \(y\) taking lower values, while any positive value, that is the \(\ln\) of something between \((1, \infty)\), enhances the probability of \(y\) taking higher values. Log-odds equal to 0 is exactly \(\ln(1)\), i.e. odds as 1:1; which means neutral effect on probability of \(y\) taking lower or higher values.
The log-odds are the key to interpret coefficient \(\boldsymbol{\beta}\). Any positive value of \(\beta\) means that there exists a positive association between \(x\) and \(y\), while any negative values indicate a negative association between \(x\) and \(y\). Values close to 0 demonstrates the lack of association between \(x\) and \(y\).
4 Likelihood
We have almost everything we need for our ordinal regression. We are only missing a final touch. Currently our logistic function outputs a vector of probabilities that sums to 1.
All of the intercepts \(\alpha_k\) along with the coefficients \(\beta_i\) are in log-cumulative-odds scale. If we apply the logistic function to the linear predictors \(\phi_k\) we get \(K-1\) probabilities: one for each \(\phi_k\)
We need a likelihood that can handle a vector of probabilities and outputs a single discrete value. The categorical distribution is the perfect candidate.
Categorical distribution is also called generalized Bernoulli distribution or multinoulli distribution.
A categorical distribution is a discrete probability distribution that describes the possible results of a random variable that can take on one of \(K\) possible categories, with the probability of each category separately specified. It is **parameterized by a vector of probabilities \(\mathbf{p}\) where each \(p_1, \dots, p_k\) is a valid probability and sums to 1:
\[p_k \geq 0, \sum^K_{k=1} p_k = 1\]
In Pumas (and, in general, in Julia) you can specify a categorical distribution using the Categorical
distribution type. By default, it takes a sequence of arguments as probabilities with the constraint that they must sum up to 1. Very similar to the “math” version.
For example here it is a categorical distribution with 4 possible discrete values and for each value probability 0.2, 0.3, 0.1, 0.4; respectively:
= [0.2, 0.3, 0.1, 0.4]
p = 1:length(p)
x = Categorical(p)
d = map(x -> pdf(d, x), x)
pdf_values data((; x, pdf_values)) *
mapping(:x => "outcome", :pdf_values => "PMF") *
visual(BarPlot) |> draw
5 Full Statistical Model
So the full statistical model with all the transformations and parametrizations we exposed so far becomes:
\[\begin{aligned} \mathbf{y} &\sim \mathrm{Categorical}(\mathbf{p}) \\ \mathbf{p} &= \mathrm{logistic}(\boldsymbol{\phi}) \\ \boldsymbol{\phi} &= \boldsymbol{\alpha} + \mathbf{X} \cdot \boldsymbol{\beta} \\ \alpha_1 &= \mathrm{CDF}(y_1) \\ \alpha_k &= \mathrm{CDF}(y_k) - \mathrm{CDF}(y_{k-1}) \quad \mathrm{for} \quad 1 < k < K-1 \\ \alpha_{K-1} &= 1 - \mathrm{CDF}(y_{K-1}) \end{aligned}\]
where:
- \(\mathbf{y}\): ordered discrete dependent variable
- \(\mathbf{p}\): probability vector of length \(K\)
- \(K\): number of possible values \(\mathbf{y}\) can take, i.e. number of ordered discrete values
- \(\boldsymbol{\phi}\): log-cumulative-odds, i.e. cut points considering the intercepts and covariates effect
- \(\alpha_k\): intercept in log-cumulative-odds for each \(k \in K-1\)
- \(\mathbf{X}\): covariate data matrix
- \(\boldsymbol{\beta}\): coefficient vector of the same length as the number of columns in \(\mathbf{X}\)
- \(\mathrm{logistic}\): logistic function
- \(\mathrm{CDF}\): cumulative distribution function
Please note that the first cut off is only \(\alpha_1\) and the last cut point is \(1 - \alpha_{\mathrm{K-1}}\).
6 pk_painrelief
Dataset
Let’s use the pk_painrelief
dataset from PharmaDatasets.jl
to run our categorical regression example in this lesson:
using PharmaDatasets
= dataset("pk_painrelief")
pk_painrelief first(pk_painrelief, 5)
Row | Subject | Time | Conc | PainRelief | PainScore | RemedStatus | Dose |
---|---|---|---|---|---|---|---|
Int64 | Float64 | Float64 | Int64 | Int64 | Int64 | String7 | |
1 | 1 | 0.0 | 0.0 | 0 | 3 | 1 | 20 mg |
2 | 1 | 0.5 | 1.15578 | 1 | 1 | 0 | 20 mg |
3 | 1 | 1.0 | 1.37211 | 1 | 0 | 0 | 20 mg |
4 | 1 | 1.5 | 1.30058 | 1 | 0 | 0 | 20 mg |
5 | 1 | 2.0 | 1.19195 | 1 | 1 | 0 | 20 mg |
We can see that we have 1920 observations for 160 different subjects and also some key variables:
:Subject
: discrete variable indicating subject ID.:Time
: continuous variable indicating time in hours.:Conc
: concentration (mg/L).:PainRelief
: discrete binary variable indicating if the subject experienced pain relief.:PainScore
(dependent variable): discrete ordinal ranging from 0 to 3.:RemedStatus
: discrete binary variable indicating if the subject has requested remedication.:Dose
: dosage of the pain medication. Either placebo, 5mg, 20mg or 80mg.
6.1 Exploratory Data Analysis
As we can see, the column :PainScore
, our dependent variable, takes values from 0 to 3. However, since the categorical distribution expects discrete values that ranges from 1 to \(\infty\), we need add 1 to every value of :PainScore
. This shifts its values, changing the range of possible values to become from 1 to 4:
|> unique |> sort pk_painrelief.PainScore
4-element Vector{Int64}:
0
1
2
3
@rtransform! pk_painrelief :PainScore = :PainScore + 1
|> unique |> sort pk_painrelief.PainScore
4-element Vector{Int64}:
1
2
3
4
Let’s explore our data with some summaries. First, we would like to know the mean and median of :PainRelief
and :PainScore
stratified by :Dose
.
We can accomplish this with a groupby()
inside @chain
macro:
Notice that we are using [:col1, :col2, ...] .=> [fun1 fun2 ...]
inside a escaped $(...)
call to the @combine
macro. This will create all the possible combinations of col
s and fun
s as summary functions to apply to our groups.
@chain pk_painrelief begin
groupby(:Dose)
@combine $([:PainRelief, :PainScore] .=> [mean median std])
@orderby :PainRelief_mean
end
Row | Dose | PainRelief_mean | PainScore_mean | PainRelief_median | PainScore_median | PainRelief_std | PainScore_std |
---|---|---|---|---|---|---|---|
String7 | Float64 | Float64 | Float64 | Float64 | Float64 | Float64 | |
1 | Placebo | 0.283333 | 2.92917 | 0.0 | 3.0 | 0.451087 | 0.772156 |
2 | 5 mg | 0.445833 | 2.58958 | 0.0 | 3.0 | 0.497576 | 0.854984 |
3 | 20 mg | 0.595833 | 2.32917 | 1.0 | 2.0 | 0.491242 | 0.804465 |
4 | 80 mg | 0.664583 | 2.16042 | 1.0 | 2.0 | 0.472628 | 0.823263 |
As we can see there is a significant correlation between :Dose
, :PainRelief
and :PainScore
.
6.2 Visualizations
Let’s do some plotting. First, we’ll explore our dependent variable: :PainScore
:
data(pk_painrelief) *
mapping(:Dose => sorter(["Placebo", "5 mg", "20 mg", "80 mg"]), :PainScore) *
expectation() |> draw AlgebraOfGraphics.
As the dose get’s higher, the perceived pain score gets lower.
Let’s talke a look at the relationship between concentration and pain relief stratified by dosage.
We need a dataset without the placebo:
= @rsubset pk_painrelief :Dose != "Placebo"; pk_painrelief_drop_placebo
data(pk_painrelief_drop_placebo) *
mapping(:Conc, :PainScore; layout = :Dose => sorter(["5 mg", "20 mg", "80 mg"])) *
visual(; linewidth = 3) *
linear() |> (x -> draw(x; facet = (; linkxaxes = :none))) AlgebraOfGraphics.
We can clearily see a negative relationship between concentration and perceived pain score.
7 Pumas modeling
Finally, we’ll create a Pumas model for ordinal regression.
7.1 Pumas’ Workflow
- Define a model. It can be a
PumasModel
or aPumasEMModel
. - Define a
Subject
andPopulation
. - Fit your model.
- Perform inference on the model’s population-level parameters (also known as fixed effects).
- Predict from a fitted model using the empirical Bayes estimate or Simulate random observations from a fitted model using the sampling distribution (also known as likelihood).
- Model diagnostics and visual predictive checks.
Pumas modeling is a highly iterative process. Eventually you’ll probably go back and forth in the stages of workflow. This is natural and expected in Pumas’ modeling. Just remember to have a good code organization and version control for all of the workflow iterations.
But first, notice that :Dose
is a string with either 5mg, 20mg, 80mg, or placebo. We need to convert the :Dose
column to a dummy variable (placebo versus treatment).
|> unique pk_painrelief.Dose
4-element Vector{InlineStrings.String7}:
"20 mg"
"80 mg"
"Placebo"
"5 mg"
@rtransform! pk_painrelief :isRx = Int(:Dose != "Placebo");
7.1.1 Defining a model in Pumas
To define a model in Pumas, you can use either the macros or the function interfaces. We will cover the macros interface in this lesson.
To define a model using the macro interface, you start with a begin .. end
block of code with @model
:
= @model begin end my_model
PumasModel
Parameters:
Random effects:
Covariates:
Dynamical system variables:
Dynamical system type: No dynamical model
Derived:
Observed:
This creates an empty model. Now we need to populate it with model components. These are additional macros that we can include inside the @model
definition. We won’t be covering all the possible macros that we can use in this lesson, but here is the full list:
@param
, fixed effects specifications.@random
, random effects specifications.@covariates
, covariate names.@pre
, pre-processing variables for the dynamic system and statistical specification.@dosecontrol
, specification of any dose control parameters present in the model.@vars
, shorthand notation.@init
, initial conditions for the dynamic system.@dynamics
, dynamics of the model.@derived
, statistical modeling of dependent variables.@observed
, model information to be stored in the model solution.
7.1.1.1 Model parameter with @param
Ok, let’s start with our ordinal_model
using the macro @model
interface.
First, we’ll define our parameters with the @parameters
macro:
@param begin
∈ Domain(...)
ParameterName ...
end
To use the “in” (∈
) operator in Julia, you can either replace ∈
for in
or type \in <TAB>
for the Unicode character.
By using the begin ... end
block we can specify one parameter per line. We will just name the parameters the same name as it’s respective covariate, while also adding the β
prefix to make clear that this is a coefficient.
Regarding the Domain(...)
, Pumas has several types of domains for you to specify in your @param
block. Here is a list:
RealDomain
for scalar parametersVectorDomain
for vectorsPDiagDomain
for positive definite matrices with diagonal structurePSDDomain
for general positive semi-definite matrices
We will only use scalar parameters in our logistic regression model, so we just need the RealDomain
.
If you don’t specify any arguments inside the domain constructor it will either error (for some domains that have required arguments) or will use the defaults. In the case of the RealDomain()
without arguments it just uses the following arguments:
RealDomain(; lower = -∞, upper = ∞, init = 0)
@param begin
# intercepts
∈ RealDomain(; init = 0.001)
α₁ ∈ RealDomain(; init = 0.002)
α₂ ∈ RealDomain(; init = 0.003)
α₃ # coefficients
∈ RealDomain()
βRx end
7.1.1.1.1 Ordered Intercepts αₖ
s
As you can see we opted for initial values very close to zero, but also they have another property: the initial values are ordered.
This makes it easier for the model to converge, and in our case not having these initial parameter values would not make the model converge at all.
7.1.1.2 Model covariates with @covariates
We also have to specify our model covariates using the macro @covariates
. This can be done using a one-liner syntax, without begin ... end
block:
@covariates Dose
Or using the begin ... end
block and specifying one covariate per line. This is what we will do, since our model has several covariates, which makes easy to visualize them and also to comment out a covariate to perform iterative model exploration:
@covariates begin
Doseend
7.1.1.3 Pre-processing variables with @pre
We can specify all the necessary variable and statistical pre-processing with the @pre
macro.
The @pre
block is traditionally used to specify the inputs of the Ordinary D ifferential Equations (ODE) system used for non-linear mixed-effects PK/PD models (NLME).
But we will use @pre
to specify our variable transformations instead.
Here, we are defining all the coefficients-covariates multiplication operation here. We are also defining our linear predictors ϕₖ
s that should be added to the intercept and passed to the non-linear logistic function transformation to become cut points cutₖ
.
Finally, we need our logistic
function. It could be tricky to use our own user-defined logistic function because it would not be numerical stable. For this, we can use the logistic
function from StatsFuns.jl
package, which Pumas reexports to us.
To use the logistic
function from StatsFuns.jl
, you just need to load Pumas:
using Pumas
# now we should have logistic available
logistic(x)
So the @pre
block becomes:
@pre begin
# linear predictors in log-cumulative-odds
= (Dose != "Placebo") * βRx
isRx
= α₁ + isRx
ϕ₁ = α₂ + isRx
ϕ₂ = α₃ + isRx
ϕ₃
# cut points for >= 1, >=2 and >=3
= logistic(ϕ₁)
cut₁ = logistic(ϕ₂)
cut₂ = logistic(ϕ₃)
cut₃
# probabilities for PainRelief (Y) =1, =2, =3 and =4
= 1.0 - cut₃
p₄ = cut₃ - cut₂
p₃ = cut₂ - cut₁
p₂ = cut₁
p₁ end
7.1.1.4 Statistical modeling of dependent variables with @derived
Our final block, **@derived
, is used to specify all the assumed distributions of observed variables that are derived from the blocks above. This is where we include our dependent variable: PainScore
**.
Note that PainScore
is being declared as following a Categorical
distribution. This is why we use the tilde notation ~
. It means (much like the mathematical model notation) that PainScore
follows a Categorical
distribution. Since PainScore
is a vector of values, we need to broadcast, i.e. vectorize, the operation with the dot .
operator:
~ Categorical.(p₁, ..., pₖ) PainScore
where p₁, ..., pₖ
are the probabilities of PainScore
taking values 1, ..., k
by subtracting the cut points. This is how we parametrize a Categorical distribution. With @.
vectorization this becomes:
~ @. Categorical(p₁, p₂, p₃, p₄) PainScore
We are using the @.
macro which tells Julia to vectorize (add the “dot syntax”) to all operations and function calls to the right of it.
In this block we can use all variables defined in the previous blocks, in our example the @param
, @covariates
and @pre
blocks.
Here is our full model, once we combined all the macro blocks we just covered:
= @model begin
ordinal_model @param begin
# intercepts
∈ RealDomain(; init = 0.001)
α₁ ∈ RealDomain(; init = 0.002)
α₂ ∈ RealDomain(; init = 0.003)
α₃ # coefficients
∈ RealDomain()
βRx end
@covariates begin
isRxend
@pre begin
# linear predictors in log-cumulative-odds
= isRx * βRx
_isRx
= α₁ + _isRx
ϕ₁ = α₂ + _isRx
ϕ₂ = α₃ + _isRx
ϕ₃
# cut points for >= 1, >=2 and >=3
= logistic(ϕ₁)
cut₁ = logistic(ϕ₂)
cut₂ = logistic(ϕ₃)
cut₃
# probabilities for PainRelief (Y) =1, =2, =3 and =4
= 1.0 - cut₃
p₄ = cut₃ - cut₂
p₃ = cut₂ - cut₁
p₂ = cut₁
p₁ end
@derived begin
~ @. Categorical(p₁, p₂, p₃, p₄)
PainScore end
end
PumasModel
Parameters: α₁, α₂, α₃, βRx
Random effects:
Covariates: isRx
Dynamical system variables:
Dynamical system type: No dynamical model
Derived: PainScore
Observed: PainScore
In this model example we have simplified some things for the sake of the tutorial. If you want a fail proof model, you’ll probably would want to add positive constraints on the pₖ
s to make sure that they are a valid probability (non-negative).
7.1.2 Define a Subject
and Population
Once we have our model defined we have to specify a Subject
and a Population
.
In Pumas, subjects (i.e. observations) are represented by the Subject
type and collections of subjects are represented as vectors of Subject
s are defined as Population
.
Subjects
can be constructed with the Subject()
constructor, for example:
Subject()
Subject
ID: 1
We just constructed a Subject
that has ID
equal to 1
and no extra information. In order to provide extra information, you would need to pass keyword arguments to the Subject()
constructor.
You can use the following keyword arguments inside Subject()
:
id
: identifier.observations
: a named tuple of the dependent variables.covariates
: a named tuple containing the covariates, ornothing
.events
: a vector ofEvent
s.time
: a vector of time stamps for the observations.
For this lesson, we will just use the covariates
keyword argument.
This is an example of a Subject
with ID
equal to 42
and with some covariates
specified as a named tuple:
Subject(id = 42, covariates = (; PainScore = 2, isRx = 0))
Subject
ID: 42
Covariates: PainScore, isRx
Since a Population
is just a vector of Subjects
, we can use a simple map()
function over the ID
s present at our nausea
dataset:
= map(
pop -> Subject(
i = i,
id = (; PainScore = pk_painrelief.PainScore, isRx = pk_painrelief.isRx),
covariates
),1:nrow(pk_painrelief),
)
Population
Subjects: 1920
Covariates: PainScore, isRx
Observations:
7.1.2.1 Reading Subject
s directly from a DataFrame
Another option is to use the read_pumas()
function to read Subject
s (and Population
) directly from a DataFrame
.
This is more convenient than the map()
with the Subject()
constructor inside.
The read_pumas()
function accepts as first argument a DataFrame
followed by the following keyword options (which are very similar to the Subject()
constructor):
observations
: dependent variables specified by a vector of column names, i.e.[:PainScore]
.covariates
: covariates specified by a vector of column names, i.e.[:Dose_5mg, :Dose_20mg, ...]
.id
: specifies theID
column of theDataFrame
.time
: specifies thetime
column of theDataFrame
.event_data
: toggles assertions applicable to event data, eithertrue
or `false.
Our pk_painrelief
dataset do not have any event data, so we should set event_data=false
.
Now, we can create our population with read_pumas()
:
= read_pumas(
pk_painrelief_pop
pk_painrelief;= [:PainScore],
observations = [:isRx],
covariates = :Subject,
id = :Time,
time = false,
event_data )
Population
Subjects: 160
Covariates: isRx
Observations: PainScore
7.1.3 Fit your model
Now we are ready to fit our model! We already have a model specified, ordinal_model
, along with a Population
: nausea_pop
. We can proceed with model fitting.
Model fiting in Pumas has the purpose of estimate parameters and is done by calling the fit()
function with the following positional arguments:
- Pumas model.
- a
Population
. - a named tuple of the initial parameter’s values.
- an inference algorithm.
7.1.3.1 Initial Parameter’s Values
We already covered model and Population
, now let’s talk about initial parameter’s values. It is the 3rd positional argument inside fit()
.
You can specify you initial parameters as a named tuple. For instance, if you want to have a certain parameter, βRx
, as having an initial value as 3.14
, you can do so by passing it inside a named tuple in the 3rd positional argument of fit()
:
fit(model, population, (; βRx = 3.14))
You can also use the helper function init_params()
which will recover all the initial parameters we specified inside the model’s @param
block.
7.1.3.2 Inference Algorithms
Finally, our last (4th) positional argument is the choice of inference algorithm.
Pumas has the following available inference algorithms:
Marginal Likelihood Estimation:
NaivePooled()
: first order approximation without random-effects.FO()
: first-order approximation.FOCE()
: first-order conditional estimation with automatic interaction detection.LaplaceI()
: second-order Laplace approximation.
Bayesian with Markov Chain Monte Carlo (MCMC):
BayesMCMC()
: MCMC using No-U-Turn Sampler (NUTS).
We can also use a Maximum A Posteriori (MAP) estimation procedure for any marginal likelihood estimation algorithm. You just need to call the MAP()
constructor with the desired marginal likelihood algorithm inside, for instance:
fit(model, population, init_params(model), MAP(FOCE()))
Ok, we are ready to fit our model. Let’s use the NaivePooled()
since we are not using random-effects in this lesson:
=
pk_painrelief_fit fit(ordinal_model, pk_painrelief_pop, init_params(ordinal_model), Pumas.NaivePooled())
[ Info: Checking the initial parameter values.
[ Info: The initial negative log likelihood and its gradient are finite. Check passed.
Iter Function value Gradient norm
0 1.230681e+04 7.478863e+05
* time: 0.04347705841064453
1 2.774236e+03 5.272884e+02
* time: 0.8824341297149658
2 2.773594e+03 5.263792e+02
* time: 0.8916590213775635
3 2.490586e+03 2.354869e+02
* time: 0.8998291492462158
4 2.427836e+03 1.586869e+02
* time: 0.9079909324645996
5 2.407966e+03 1.142587e+02
* time: 0.915539026260376
6 2.406268e+03 1.174064e+02
* time: 0.9230799674987793
7 2.406173e+03 1.177969e+02
* time: 0.930851936340332
8 2.406095e+03 1.177489e+02
* time: 0.9383101463317871
9 2.405790e+03 1.167671e+02
* time: 0.9455780982971191
10 2.405120e+03 1.134714e+02
* time: 1.047055959701538
11 2.403414e+03 1.034002e+02
* time: 1.052631139755249
12 2.399807e+03 8.350230e+01
* time: 1.0582630634307861
13 2.393612e+03 8.371963e+01
* time: 1.063857078552246
14 2.387107e+03 6.956438e+01
* time: 1.0693869590759277
15 2.384192e+03 5.506164e+01
* time: 1.0747020244598389
16 2.383684e+03 5.470704e+01
* time: 1.0801129341125488
17 2.383628e+03 4.934203e+01
* time: 1.0855889320373535
18 2.383620e+03 4.820876e+01
* time: 1.0908210277557373
19 2.383580e+03 4.460237e+01
* time: 1.097222089767456
20 2.383495e+03 3.989466e+01
* time: 1.1042590141296387
21 2.383255e+03 3.945416e+01
* time: 1.1112380027770996
22 2.382653e+03 4.049661e+01
* time: 1.1183271408081055
23 2.381116e+03 4.113760e+01
* time: 1.1256849765777588
24 2.377501e+03 6.052250e+01
* time: 1.172605037689209
25 2.370218e+03 9.150939e+01
* time: 1.1781351566314697
26 2.360024e+03 1.181281e+02
* time: 1.183506965637207
27 2.352292e+03 1.177431e+02
* time: 1.1889419555664062
28 2.349688e+03 9.926825e+01
* time: 1.1944279670715332
29 2.349433e+03 8.973818e+01
* time: 1.1998181343078613
30 2.349410e+03 8.801720e+01
* time: 1.2055189609527588
31 2.349370e+03 8.603872e+01
* time: 1.2110049724578857
32 2.349276e+03 8.310529e+01
* time: 1.2162611484527588
33 2.349025e+03 7.793090e+01
* time: 1.2226850986480713
34 2.348392e+03 6.897156e+01
* time: 1.2292449474334717
35 2.346829e+03 5.322713e+01
* time: 1.2362110614776611
36 2.343358e+03 5.614960e+01
* time: 1.2427959442138672
37 2.337197e+03 5.563204e+01
* time: 1.2494730949401855
38 2.330256e+03 3.881416e+01
* time: 1.2867951393127441
39 2.326721e+03 1.838918e+01
* time: 1.2925641536712646
40 2.326173e+03 4.369402e+00
* time: 1.29823899269104
41 2.326149e+03 3.957062e-01
* time: 1.3038809299468994
42 2.326148e+03 3.741394e-02
* time: 1.3095719814300537
43 2.326148e+03 1.798433e-03
* time: 1.315222978591919
44 2.326148e+03 5.160531e-05
* time: 1.3208630084991455
FittedPumasModel
Successful minimization: true
Likelihood approximation: NaivePooled
Likelihood Optimizer: BFGS
Dynamical system type: No dynamical model
Log-likelihood value: -2326.148
Number of subjects: 160
Number of parameters: Fixed Optimized
0 4
Observation records: Active Missing
PainScore: 1920 0
Total: 1920 0
-----------------
Estimate
-----------------
α₁ -3.0216
α₂ -0.89655
α₃ 1.0973
βRx 1.2369
-----------------
7.1.4 Perform inference on the model’s population-level parameters
Once the model is fitted, we can analyze our inference and estimates. But first, let’s discuss what the is the meaning of the estimated coefficients in a ordinal regression model.
7.1.4.1 Interpreting the Coefficients
We can see that after the model is fitted, it prints a result with a summary and a table of the parameter estimates.
We can also recover the estimates as a named tuple with coef()
:
coef(pk_painrelief_fit)
(α₁ = -3.021616028925512,
α₂ = -0.8965453588452162,
α₃ = 1.0972549105426705,
βRx = 1.2368716303859093,)
Or as a DataFrame
with coeftable()
:
coeftable(pk_painrelief_fit)
Row | parameter | estimate |
---|---|---|
String | Float64 | |
1 | α₁ | -3.02162 |
2 | α₂ | -0.896545 |
3 | α₃ | 1.09725 |
4 | βRx | 1.23687 |
Remember that the ordinal regression’s coefficients are the log-cumulative-odds of the cut points, i.e. the natural logarithm of the odds of \(y\) taking values less or equal to \(k\).
Log-cumulative-odds provide the facility that anything that is negative pulls down the odds (and, consequently, the probability) of \(y\) taking higher values. Anything positive pulls it up; and zero is a neutral effect. But since it is in a log-scale it is not easily interpretable. This is why, in order for interpret it, often we undo the log transformation by exponentiating the log-cumulative-odds, i.e. exponentiating the coefficients.
We can do that easily with the DataFrame
returned by coeftable()
:
@rtransform coeftable(pk_painrelief_fit) :estimate_exp = exp(:estimate)
Row | parameter | estimate | estimate_exp |
---|---|---|---|
String | Float64 | Float64 | |
1 | α₁ | -3.02162 | 0.0487224 |
2 | α₂ | -0.896545 | 0.407977 |
3 | α₃ | 1.09725 | 2.99593 |
4 | βRx | 1.23687 | 3.44482 |
Now let us interpret the :estimate_exp
column.
Our basal rates αₖ
s are log-cumulative-odds of the \(k\) cut points, i.e. the odds of \(y\) taking value less or equal than \(k\). For example α₁
has odds approximate to 0.05. That means that the odds of \(y \leq 1 = 0.05\).
Ordinal regression, like linear and logistic regression, has the assumption that the effects are a linear combination. That means that a prediction can be made by having the basal rates αₖ
s and keep adding covariates values multiplied their β
coefficients. This assumption also holds for parameter/model inference. Hence, all of our coefficients represent compounding effects into our dependent variable \(y\).
What we accomplish with the basal rates αₖ
s is just that we can violate the equidistant assumption.
As an example for the interpretation of coefficients, let us interpret the βRx
: the covariate isRx
’s treatment (non-placebo) coefficient. It’s value is approximate 3.44, which means that for treatment we can expect an increase in 2.44x in the odds of having higher values of perceived pain relief against placebo.
If you ever heard of odds ratio or relative risk, this is exactly what we are measuring here with our exponentiated log-cumulative-odds. It measures the odds ratio between having a dependent variable higher values over having lower values.
We can also inspect our estimated coefficients’ standard errors (SE
) along with the 95% confidence intervals with the infer()
function:
infer(pk_painrelief_fit)
[ Info: Calculating: variance-covariance matrix.
[ Info: Done.
Asymptotic inference results using sandwich estimator
Successful minimization: true
Likelihood approximation: NaivePooled
Likelihood Optimizer: BFGS
Dynamical system type: No dynamical model
Log-likelihood value: -2326.148
Number of subjects: 160
Number of parameters: Fixed Optimized
0 4
Observation records: Active Missing
PainScore: 1920 0
Total: 1920 0
----------------------------------------------------------
Estimate SE 95.0% C.I.
----------------------------------------------------------
α₁ -3.0216 0.20597 [-3.4253 ; -2.6179 ]
α₂ -0.89655 0.19315 [-1.2751 ; -0.51798]
α₃ 1.0973 0.19705 [ 0.71104; 1.4835 ]
βRx 1.2369 0.21954 [ 0.80659; 1.6672 ]
----------------------------------------------------------
Also if you prefer other confidence interval band, you can choose with the keyword argument level
inside infer()
.
For instance, one common band for the confidence intervals are 89%:
infer(pk_painrelief_fit; level = 0.89)
[ Info: Calculating: variance-covariance matrix.
[ Info: Done.
Asymptotic inference results using sandwich estimator
Successful minimization: true
Likelihood approximation: NaivePooled
Likelihood Optimizer: BFGS
Dynamical system type: No dynamical model
Log-likelihood value: -2326.148
Number of subjects: 160
Number of parameters: Fixed Optimized
0 4
Observation records: Active Missing
PainScore: 1920 0
Total: 1920 0
----------------------------------------------------------
Estimate SE 89.0% C.I.
----------------------------------------------------------
α₁ -3.0216 0.20597 [-3.3508 ; -2.6924 ]
α₂ -0.89655 0.19315 [-1.2052 ; -0.58785]
α₃ 1.0973 0.19705 [ 0.78233; 1.4122 ]
βRx 1.2369 0.21954 [ 0.88601; 1.5877 ]
----------------------------------------------------------
7.1.5 Predict from a fitted model or Simulate random observations from a fitted model
Now that we understand our model estimates, we can use it to make predictions or simulate random observations.
7.1.5.1 Predictions with predict()
In order to calculate prediction from a fitted model, we need to use the predict()
function and passing two positional arguments:
- a fitted Pumas model.
- either a single
Subject
or a collection of them with aPopulation
.
For example to make predictions onto a single Subject
, we can:
= Subject(id = 42, covariates = (; PainScore = 2, isRx = 0)) my_subject
Subject
ID: 42
Covariates: PainScore, isRx
predict()
also needs a keyword argument obstimes
which represents the observation times that we want to predict the dependent variable. The value must be a vector. Since we used a dummy 0.0
value as :time
variable in our read_pumas
function, we can pass the same 0.0
but as a vector to the predict()
function.
predict(pk_painrelief_fit, my_subject; obstimes = [0.0])
SubjectPrediction
ID: 42
Predictions: PainScore: (n=1)
Covariates: PainScore, isRx
Time: [0.0]
We can also generate prediction for a Population
:
predict(pk_painrelief_fit, pk_painrelief_pop; obstimes = [0.0])
Prediction
Subjects: 160
Predictions: PainScore
Covariates: isRx
We can also use the function probstable()
for discrete models. It provides an easy and elegant way of outputting outcome probabilities of all discrete dependent variables in a handy DataFrame
format.
See below, that we have the :PainScore_iprob1
up to :PainScore_iprob4
columns. These columns take the following formatting: :DEPVAR_iprobVALUE
. Since PainScore
can take four discrete outcomes, i.e. it can take values from 1 to 4, :PainScore_iprob1
maps to PainScore
taking value 1 and so on.
= probstable(pk_painrelief_fit)
probs_table first(probs_table, 5)
Row | id | time | PainScore_iprob1 | PainScore_iprob2 | PainScore_iprob3 | PainScore_iprob4 |
---|---|---|---|---|---|---|
String | Float64 | Float64 | Float64 | Float64 | Float64 | |
1 | 1 | 0.0 | 0.143718 | 0.440551 | 0.327394 | 0.0883358 |
2 | 1 | 0.5 | 0.143718 | 0.440551 | 0.327394 | 0.0883358 |
3 | 1 | 1.0 | 0.143718 | 0.440551 | 0.327394 | 0.0883358 |
4 | 1 | 1.5 | 0.143718 | 0.440551 | 0.327394 | 0.0883358 |
5 | 1 | 2.0 | 0.143718 | 0.440551 | 0.327394 | 0.0883358 |
7.1.6 Simulations with simobs()
We can generate random observations from our fitted Pumas model with the simobs()
function. You need to supply at least three positional arguments:
- a non-fitted Pumas model, in our case
ordinal_model
. - either a single
Subject
or a collection of them with aPopulation
. - a named tuple of parameter values.
The 1st and 2nd positional arguments we already covered in our previous examples. Let’s focus on the 3rd positional argument: param
. It can be a named tuple with the parameter values. For instance, we can use our estimated parameters from the fitted model with coef(nausea_fit)
:
coef(pk_painrelief_fit)
(α₁ = -3.021616028925512,
α₂ = -0.8965453588452162,
α₃ = 1.0972549105426705,
βRx = 1.2368716303859093,)
simobs(ordinal_model, pk_painrelief_pop, coef(pk_painrelief_fit));
You can also pass a single parameter as a Pumas fitted model to simobs()
.
= simobs(pk_painrelief_fit); pk_painrelief_sim
7.1.7 Model diagnostics
Finally, our last step is to assess model diagnostics.
7.1.7.1 Assessing model diagnostics
To assess model diagnostics we can use the inspect()
function in our fitted Pumas model:
inspect(pk_painrelief_fit)
[ Info: Calculating predictions.
[ Info: Calculating empirical bayes.
[ Info: Evaluating individual parameters.
[ Info: Evaluating dose control parameters.
[ Info: Done.
FittedPumasModelInspection
Fitting was successful: true
7.1.7.1.1 AIC
We can also check for Akaike Information Criterion (AIC):
aic(pk_painrelief_fit)
4660.296063423193
7.1.7.1.2 BIC
Besides AIC, there is also the Bayesian Information Criterion (BIC):
bic(pk_painrelief_fit)
4682.536385283281
7.2 Visual predictive checks
To conclude, we can inspect visual predictive checks with the vpc_plot()
function. But first, we need to generate a VPC
object with the vpc()
function.
By default, vpc
will use :time
as the independent variable for VPC. We need to change that, because or :time
variable is just a vector of 0
s in the ordinal regression.
Let’s use :isRx
:
= vpc(pk_painrelief_fit; covariates = [:isRx]); pk_painrelief_vpc
Now, we need to use the vpc_plot
function into our newly created VPC
object. For this, we need to import the package PumasUtilities
:
using PumasUtilities
= vpc_plot(pk_painrelief_vpc; paginate = true, separate = true); plots
1] plots[
4] plots[
2] plots[
3] plots[
8 Conclusion
I hope you enjoyed this tutorial on ordinal regression. Whenever your dependent variable is ordinal, there’s a great chance that it violates the equidistant assumption. This is where ordinal regression comes to rescue. Keep it close by in your arsenal of discrete statistical modeling techniques.